AI Sales Forecasting - Predict the Future and Optimize Your Sales Strategy
According to Gartner’s 2024 survey of sales operations leaders, sales forecasting has become increasingly more difficult and less precise in recent years. Despite having access to more data than ever before, companies are not seeing significant improvements in their ability to predict future sales. Nowadays, their sales forecasting accuracy achieves 60-79%, which is not nearly good enough for reliable decision-making.
However, there is a better way. The new AI sales forecasting technology allows sales managers to achieve much better precision by analyzing large datasets and interpreting complex patterns to predict future sales trends. With the help of this AI solution, businesses can derive data-driven insights to make more informed decisions about their sales processes.
In this article, we’ll discuss the benefits of AI sales forecasting and how to implement it to achieve the best results.
Why is Sales Forecasting Important?
Accurate sales forecasts help companies plan their resources, optimize sales, and adjust business strategies. With valuable insights obtained from sales forecasting, companies can ensure they have the right amount of inventory, workforce, and capital to meet future demand.
Sales forecasts are also essential for setting realistic revenue goals and allocating budgets to the areas with the highest potential. For example, a forecast can predict that one line of products will soon become popular based on current market trends and social media activity. To respond to this change in demand, a company can redirect some of the production and marketing budget from less popular products. As a result, the company can better meet customer needs and maximize sales opportunities.
Challenges of traditional sales forecasting methods
Traditional sales forecasting relies on data analysts or systems to process vast amounts of business data and extract actionable insights. However, neither conventional forecasting systems nor manual analysis can efficiently handle such large datasets or uncover the complex patterns and correlations hidden within them. This limits the accuracy and depth of insights that traditional methods can achieve.
Another challenge is the static nature of traditional forecasting methods. These systems typically use fixed models, such as moving averages, which don’t adapt fast enough to new data. As a result, they may overlook emerging trends or sudden changes in customer demand, requiring frequent manual updates and adjustments.
These limitations make traditional sales forecasting less accurate and slower to respond to market changes.
How Does AI Boost Sales Forecasting?
AI sales forecasting is a machine learning algorithm that quickly analyzes and learns from vast amounts of data, including market trends, customer behavior, historical data, social media sentiment, economic indicators, etc., to predict future sales with a maximum degree of accuracy. Unlike traditional forecasting, an AI sales forecasting model constantly adapts to new information, uncovering hidden patterns and making predictions in real-time.
It's important to recognize that AI can’t completely replace humans in the sales forecasting process. Instead, it enhances their ability to make more informed decisions about their business by delivering accurate forecasts through advanced data analysis.
Here’s a list of the main AI sales forecasting use cases:
- Product/service demand prediction - anticipates which products/services will be in high demand in the next months based on historical sales, economic trends, seasonality, etc.
- Product launch planning - helps businesses make data-driven decisions on pricing, distribution channels, marketing campaigns, etc. based on market trends, competitive analysis, historical data of similar products, etc.
- Inventory management - helps determine the right amount of products based on future demand and avoid overstocking or understocking.
- Pricing strategy - predicts how price changes might affect sales, helping companies optimize their prices to achieve maximum profitability.
- Revenue forecasting - helps sales representatives accurately predict future income, make more informed financial decisions, and set realistic growth targets.
- Cross-selling and upselling opportunities - by analyzing customer purchase history and buying patterns helps the sales team identify opportunities for cross-selling or upselling.
Benefits of AI in Sales Forecasting
AI in sales forecasting brings several benefits that empower businesses to improve their sales performance and operate more effectively:
More accurate and reliable forecasts
AI algorithms excel at identifying complex patterns and trends that traditional forecasting methods may overlook. As AI sales forecasting software continuously learns from new data, they become more accurate and reliable over time.
Enhanced data-driven decision-making
AI sales forecasting provides deep and accurate insights, helping business leaders make more informed decisions. By leveraging AI forecasting capabilities, businesses can optimize sales strategies and improve their sales performance.
Increased business agility
AI’s ability to provide real-time insights and adapt to changing conditions enables businesses to be more agile. Companies can quickly respond to changes in customer demand, ensuring they are one step ahead of their competitors, ready to seize new opportunities.
Challenges in AI Sales Forecasting
While AI sales forecasting offers significant benefits, it also comes with challenges that can affect its accuracy. Here are 2 main factors that affect AI’s ability to predict future sales:
Data quality
The AI algorithm requires high-quality, accurate data to provide reliable sales forecasts. Inconsistent, incomplete, or outdated data can disrupt the algorithm's ability to predict future sales. That’s why businesses must provide access to accurate data sources such as customer behavior, feedback, market trends, etc.
Solution
To collect more comprehensive customer data, businesses can use an AI tool called activity intelligence. Activity intelligence tracks client interactions across different platforms, such as emails, calendars, meetings, and so on, without manual input. It automatically logs all customer interactions with the company to provide a complete overview of customer engagement. To further enrich the dataset used by AI sales forecasting software, businesses can subscribe to external data provider services to gain access to up-to-date information covering everything from economic forecasts to competitor insights.
Before feeding data into AI models, businesses need to make sure their data is well prepared. This means removing duplicates, filling in missing information, and correcting errors. These tasks can be automated with data-cleaning tools to ensure a seamless flow of high-quality data. Businesses should also conduct regular data audits to ensure that the AI algorithm is working with accurate and relevant information.
AI model transparency
AI sales forecasting tools can sometimes act as "black boxes". They don’t provide access to their algorithms, making it difficult for users to understand how predictions are generated. Because of this lack of transparency, sales teams may struggle to explain to their shareholders why they should trust AI-driven forecasts.
Solution
One of the solutions to this challenge is to choose an AI sales forecasting system that prioritizes transparency A perfect example is CRM Creatio, which provides a clear overview of its AI-driven forecasts.
Creatio AI sales forecasting system explains how predictions are made, ensuring that users can understand the factors and relations between them that are influencing the outcome. This transparency helps users understand how the AI reached its conclusions and trust the results.
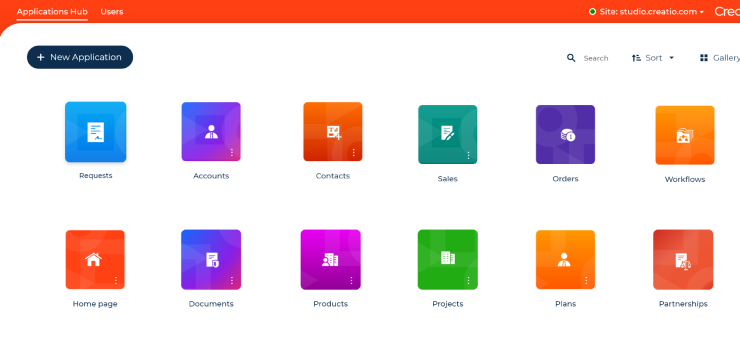
How to Implement AI Sales Forecasting?
According to Gartner, 89% of surveyed organizations already use AI-based predictive analytics, however, only 1% of sales leaders achieve forecast accuracy surpassing 95%. This indicates, that while the adoption of AI tools is widespread, organizations still struggle to take full advantage of this technology. One of the reasons for that is poorly executed implementation of AI sales forecasting systems.
By following these steps, you can ensure the best-fit forecasting system for your business case:
Step 1. Set clear goals
Identify clear goals you want to achieve with AI sales forecasting. It can be a range of objectives, from increasing the accuracy of the predictions, improving the decision-making process, optimizing inventory management, etc. Setting clear goals at the beginning will help you measure whether the implementation was successful.
Step 2. Choose the right AI sales forecasting solution
Compare vendors' offerings and make sure you find the one that meets your unique business needs. Look for solutions that offer advanced analytics capabilities, user-friendly interfaces, and transparent AI models.
Step 3. Prepare your data
To ensure that the algorithm has access to up-to-date, accurate customer information and historical sales data, integrate your forecasting solution with core business software, such as CRMs, ERPs, etc. Make sure the data is clean and complete. To further increase the accuracy of sales forecasting, subscribe to market research sources that will provide all necessary information about external factors that may impact your sales.
Step 4. Train your AI model
Build models tailored to your business by training AI on your historical data. Training may involve using one of the machine learning techniques such as linear regression, which analyzes the relationship between sales and one independent variable, or more advanced methods like neural networks, which explore nonlinear relationships in data. If you are not an AI specialist or a data scientist, make sure your vendor provides adequate support. You can also invest in a no-code tool to easily train your AI model without requiring technical expertise.
Step 5. Test the AI sales forecasting accuracy
Before deploying the model, test it to make sure it’s accurate and reliable. To do this, compare the model's predictions against the actual sales outcomes from the past. This will help you see how well the model performs and whether its analysis can be trusted. Gather the feedback from your sales reps and other stakeholders and retrain the model if necessary.
Step 6. Integrate with business processes
Integrate the AI sales forecasting model into business processes, such as developing sales strategy, inventory management, etc. Train your employees so they can interpret and act on the data to optimize their work.
Conclusions
AI-powered sales forecasting is set to dramatically improve businesses' ability to predict future sales, surpassing 95% accuracy in some cases. Compared to traditional methods, it also offers deeper insights via multi-factor analysis in real-time, helping businesses make more informed decisions here and now. On top of more accurate sales prediction data, AI sales forecasting plays a key role in improving inventory management, pricing strategy, marketing campaign planning, and other revenue-driving activities. While there are initial challenges, such as data quality and model transparency, businesses that effectively implement AI sales forecasting can gain a future-proof competitive edge over their rivals.