What is Predictive AI - The Future of Smarter Business Decisions
Predictive AI is becoming a crucial part of business decision-making, with MIT forecasting that investments in predictive models will reach $64 billion by the end of 2025.
Companies across industries leverage predictive AI insights to make data-driven decisions. In this article, we will explore what predictive AI is, how it works, and how companies can use it to optimize their operations and boost revenue growth.
What is Predictive AI?
Predictive artificial intelligence leverages machine learning and statistical analysis to analyze historical and real-time data. By identifying patterns and trends in data, predictive AI forecasts future events, outcomes, and behaviors, providing valuable insights that support informed business decisions.
Traditional predictive analytics methods rely on predefined statistical techniques and human-designed rules. These methods are often prone to error and can only be manually adjusted. As a result, the predictions are less accurate and less adaptable to changing conditions.
On the other hand, predictive AI can continuously learn from new data, adapt its models, and improve the accuracy of its predictions over time without requiring humans to manually adjust the model’s or parameters.
Predictive AI systems can provide insights regarding:
- product demand and future sales based on customer behavior, market trends, and historical sales,
- customer behavior, such as churn risk and purchasing patterns,
- next best action (NBA) recommendations that support sales and service agents in more efficient deal and case closing,
- next best offer (NBO) recommendations that suggest personalized products and highlights cross-selling and upselling opportunities,
- likelihood of closing a deal based on leads data and historical sales,
- upcoming market trends based on online buzz, demand changes, and economic shifts,
- potential supply chain disruptions due to political situations or weather changes,
- machine failure based on the wear of individual parts and time since the last service.
How Does Predictive AI Work?
As mentioned above, predictive AI analyzes historical and real-time data to derive valuable insights. The process involves data collection and preparation, model training, and continuous refinement, which is crucial to improve the accuracy of insights.
Here’s the step-by-step process:
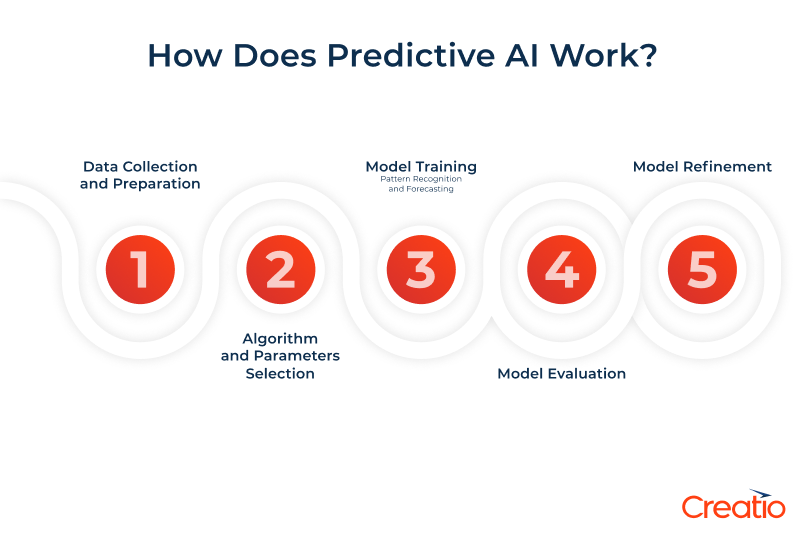
1. Data collection and preparation
Predictive AI analyzes both structured and unstructured data, such as text, images, and documents, such as:
- customer behavior (purchase history, spending habits, past interactions, website visits)
- financial data (financial reports, financial transactions, cash flow reports)
- equipment data (equipment performance reports, servicing reports, images of crucial machinery parts)
- external factors (weather conditions, inflation, political unrest, social media sentiment)
The data is typically divided into two sets - training data, which is used to teach the model, and test data, which is used to evaluate the model's performance after training.
2. Algorithm and parameters selection
In the next step, the predictive AI model is trained on existing data using machine learning algorithms chosen by data scientists. The model is trained either through supervised learning with historical data labeled by humans or through unsupervised learning, where the model identifies patterns on its own.
Common ML algorithms include:
- Regression models
- Decision trees
- Random forests
- Neural networks & deep learning
- K-means clustering
- Linear and logistic regression
- Support vector machines
After choosing the most appropriate algorithm, data scientists must select the model parameters. For example, in a neural network, this means choosing the starting values for the weights (the values that decide how much each feature matters).
3. Model training - pattern recognition and forecasting
During training, the predictive AI model analyzes provided data sets to find relationships and patterns, using its current parameters. Then, it compares its predictions to the actual values and adjusts its parameters to improve future predictions.
4. Model evaluation
Once the model has been trained, its accuracy is evaluated using test data. The goal is to see how well the model can make predictions using new data that wasn’t used to train the model.
5. Model refinement
The model can refine itself as new data becomes available. Predictive AI constantly updates its parameters and structure to improve prediction accuracy.
Predictive AI Use Cases
In this section, we will provide a few use cases of predictive AI across sales, marketing, and customer service departments. We will also explore how insights provided by predictive AI can be used in the finance and retail industry. As an example, we will use Creatio.ai, one of the most comprehensive AI platforms to automate workflows and CRM on the market.
Sales
Predictive AI can support sales teams by prioritizing leads, providing the next best action and next best offer recommendations, and forecast future sales.
Lead and opportunity scoring
With Creatio.ai, businesses can estimate the likelihood of successful sales for each lead based on the leads’ parameters and their engagement history. The insights help sales professionals prioritize leads and focus their efforts on high-potential opportunities.
Additionally, sales reps can predict the likelihood of closing a deal based on previously closed and lost deals. Thanks to opportunity scoring capabilities, predictive AI helps sales teams better understand which deals to prioritize and where to allocate resources.
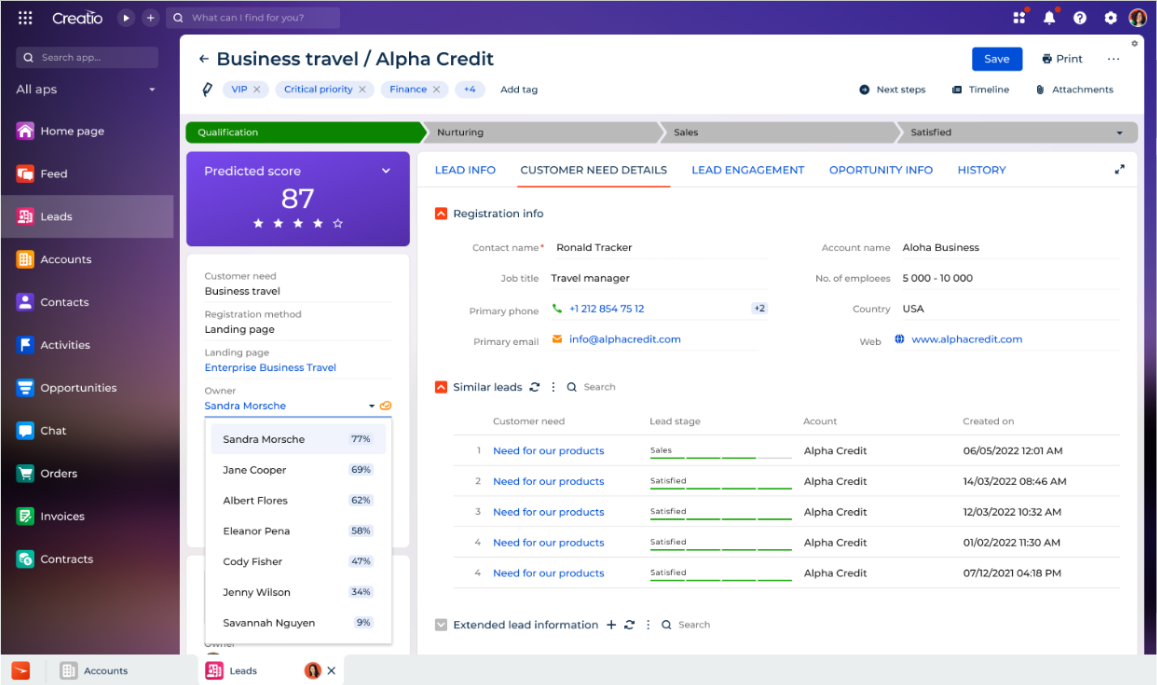
Next best action
Creatio.ai can analyze leads’ information and historical data regarding similar leads to recommend the next best step to convert the lead faster. This may include suggestions to send additional materials, schedule a call, offer a discount, etc.
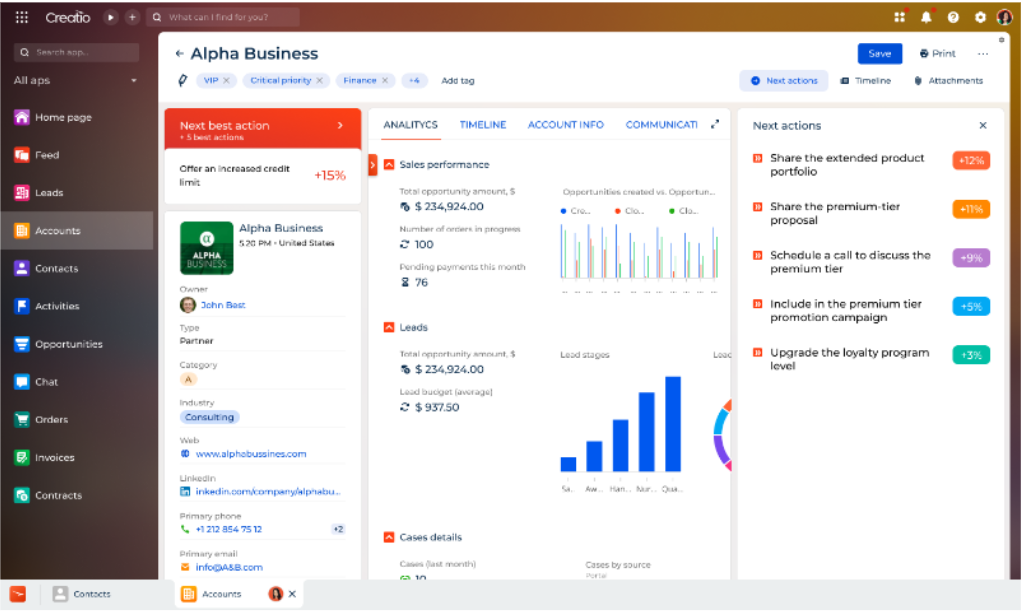
Sales forecasting
Creatio.ai uses predictive AI to predict future sales based on historical sales data. Thanks to AI-powered sales forecasting insights, businesses can more accurately predict revenue, allocate resources, manage supply chain operations, and optimize inventory levels.
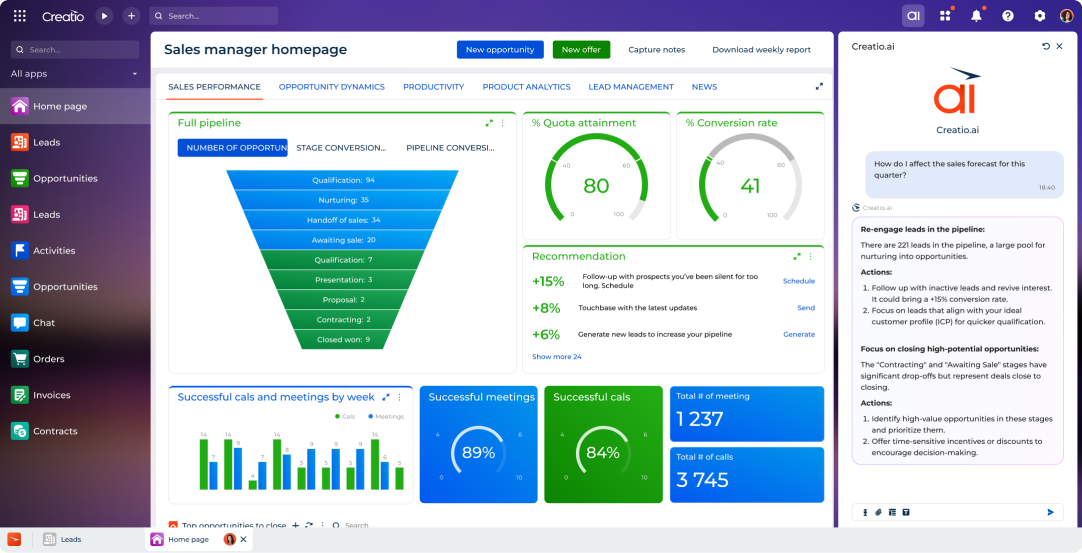
Marketing
Marketing professionals can benefit from predictive AI’s capabilities to segment a company’s audience, predict marketing campaigns success rate, and automatically recommend products based on customer needs.
Audience segmentation
Creatio.ai analyzes vast amounts of data to segment the audience into groups that can potentially respond positively to similar marketing materials and offers. Predictive AI segments the audience based on preferences, browsing habits, and previous engagement with marketing materials, enabling marketers to prepare targeted campaigns and ads.
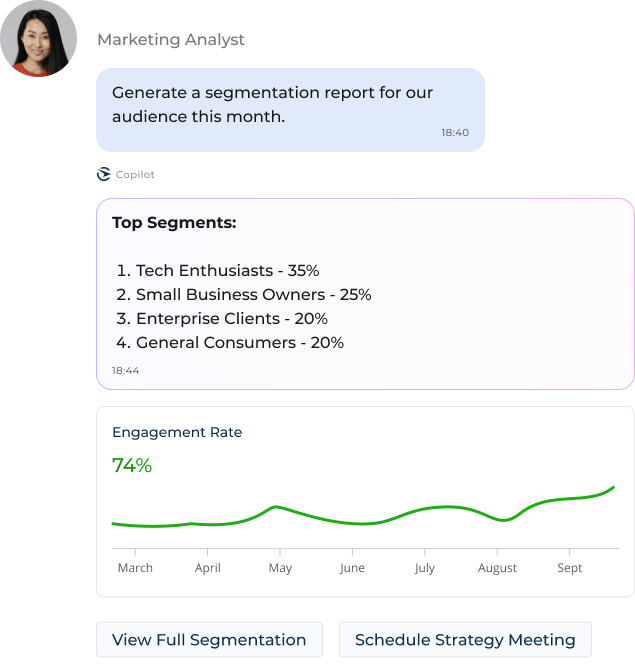
Additionally, predictive AI continuously updates audience segments in real-time as soon as new data is available. This is especially useful for accurate ad and email marketing targeting.
Campaign response and success rate prediction
Marketing professionals can use predictive AI to forecast the potential response to marketing campaigns. Creatio.ai can provide insights into how different audience segments might react to specific marketing efforts by analyzing historical data, customer behavior, and market trends.
With Creatio.ai, marketers can identify the most responsive audience segments, personalize messaging to fit their preferences, and allocate marketing budget to the channels that are most likely to generate high returns. By leveraging these insights, marketing teams can increase engagement, improve conversion rates, and maximize ROI.
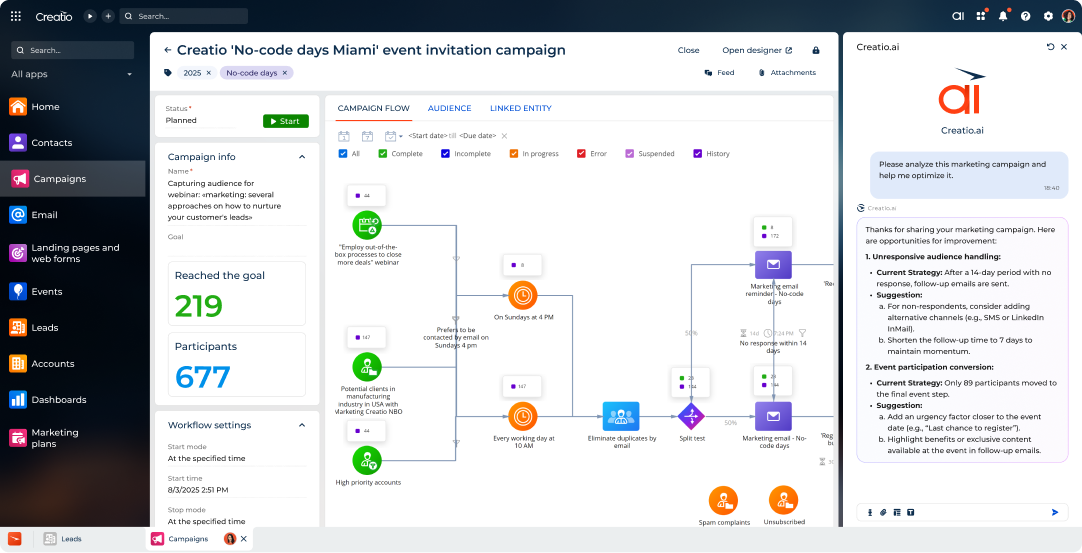
Intelligent product recommendation
Predictive AI tools analyze customer online behavior and past purchases to deliver personalized product recommendations tailored to individual customers’ needs. Creatio.ai can predict which products or services will be the most interesting for each customer and continuously adapt these recommendations to reflect changes in their preferences.
Customer service
Customer service teams can use predictive AI to prioritize cases, predict the likelihood of customer churn, and choose the next action based on the analysis of similar cases.
Service queue prioritization
Creatio.ai helps customer service agents increase their productivity by automatically prioritizing and routing cases to the most appropriate agents based on their workload and experience. Thanks to predictive AI analysis, no time-sensitive and important cases will fall through the cracks.
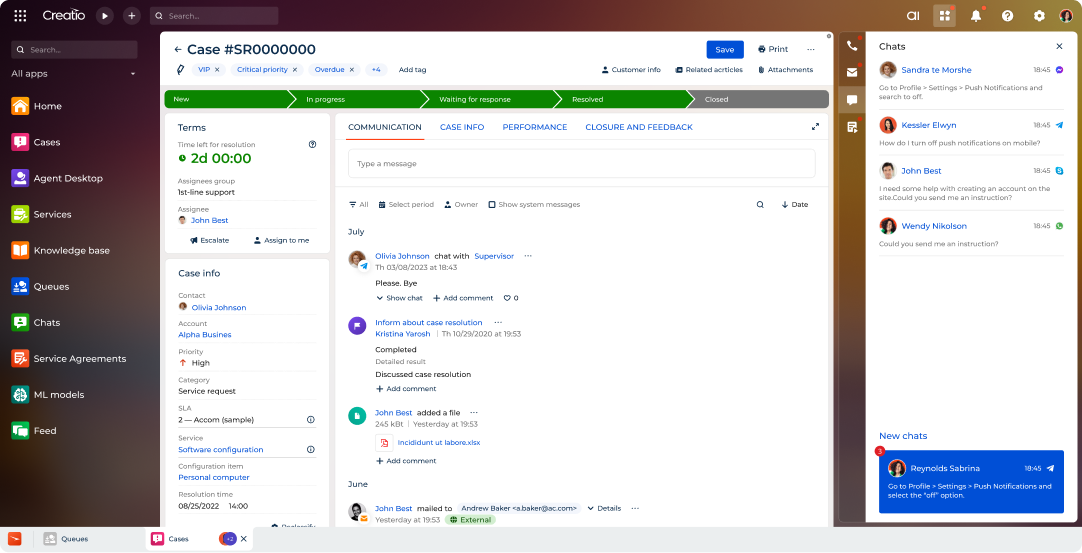
Customer sentiment and churn probability
Creatio.ai can analyze customer behavior and sentiment in real time to alert customer service agents about customers at risk of churning. By tracking their engagement, past interactions, and feedback, the system identifies early warning signs, such as reduced activity or negative sentiment. This allows agents to reach out to these customers with tailored support, personalized offers, or retention strategies to improve customer satisfaction and loyalty.
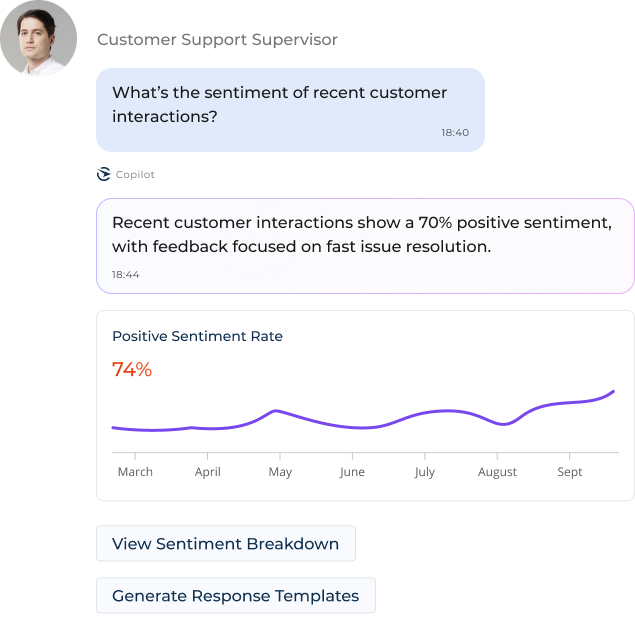
Search for similar cases
Predictive AI tools can help agents resolve cases faster and more effectively by analyzing past cases and identifying the best solutions. Creatio.ai searches the database for similar cases, learns from previous resolutions, and suggests the most effective next steps. Thanks to predictive AI, customer service agents can reduce resolution time and boost customer satisfaction.
Finance
Financial institutions can enhance their security and increase revenue thanks to insights provided by predictive AI tools.
Credit scoring
Creatio.ai can analyze a customer’s financial history, payment behavior, and even non-traditional metrics, such as social media activity, to assess customers' reliability and creditworthiness. This allows lenders to make more accurate lending decisions and offer fairer and more inclusive lending opportunities.
Fraud detection
Financial institutions can improve their fraud detection by implementing predictive AI into the process. AI can continuously monitor transactions to detect suspicious activities and unusual patterns in real time, identifying potential threats much sooner than humans. This helps recognize phishing attacks, money laundering activities, and unauthorized transactions.
By updating its database and refining prediction models, AI can quickly adapt to new types of cyber threats, increasing the overall security of financial institutions.
Retail
Retail companies can use predictive AI to forecast demand, improve the supply chain, and optimize stock levels.
Demand forecasting
Predictive AI forecasts future product demand by analyzing sales trends, seasonal patterns, and customer behavior. By accurately forecasting demand, predictive AI tools help businesses plan their inventory, preventing stockouts that lead to lost sales and overstocking that increases storage costs.
Additionally, marketing professionals can use these insights to plan marketing campaigns and prepare personalized product recommendations that accurately reflect customer demand.
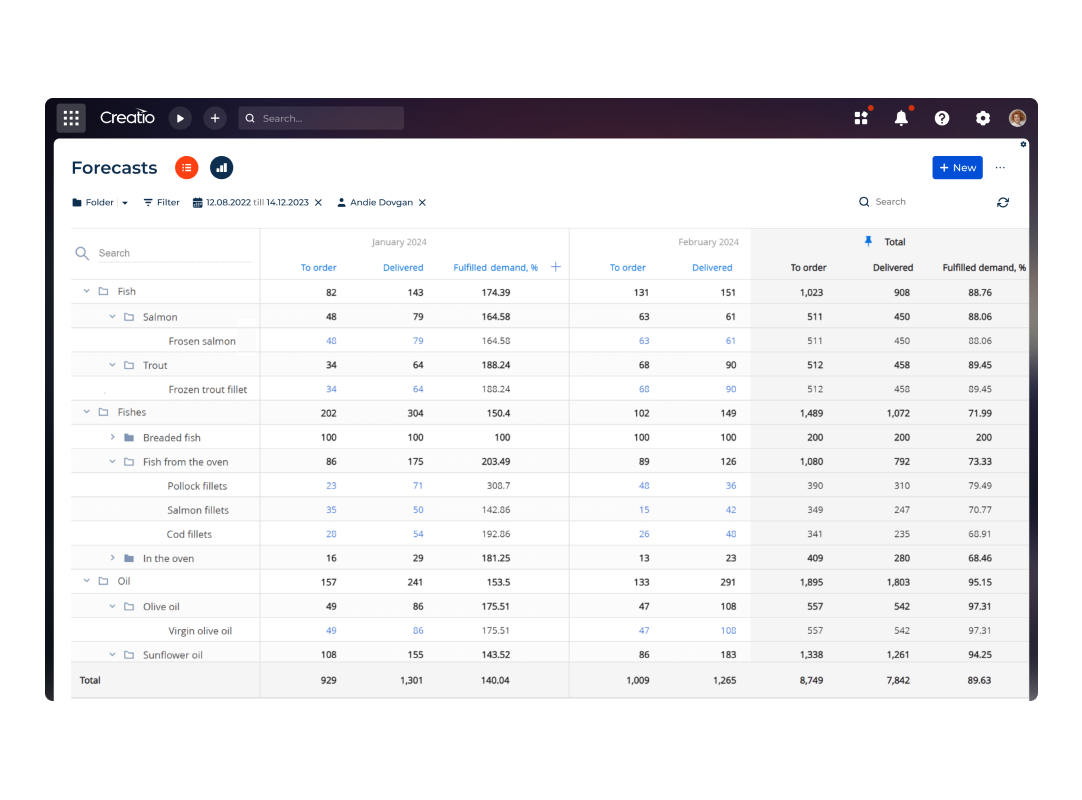
Price optimization
Creatio.ai allows retailers to dynamically optimize their pricing strategy for different products, customer segments, and distribution channels. With comprehensive sales, pricing, and profitability analytics and AI-driven predictive modeling techniques, predictive AI can help adjust prices to customer demand, competitor pricing, and customer preferences.
Supply chain optimization
Retail companies can use predictive AI insights to optimize their supply chains. By forecasting demand fluctuations, predicting delays, and recommending optimal stock levels, predictive AI allows retailers to adjust procurement and logistics to reduce costs and improve fulfillment efficiency.
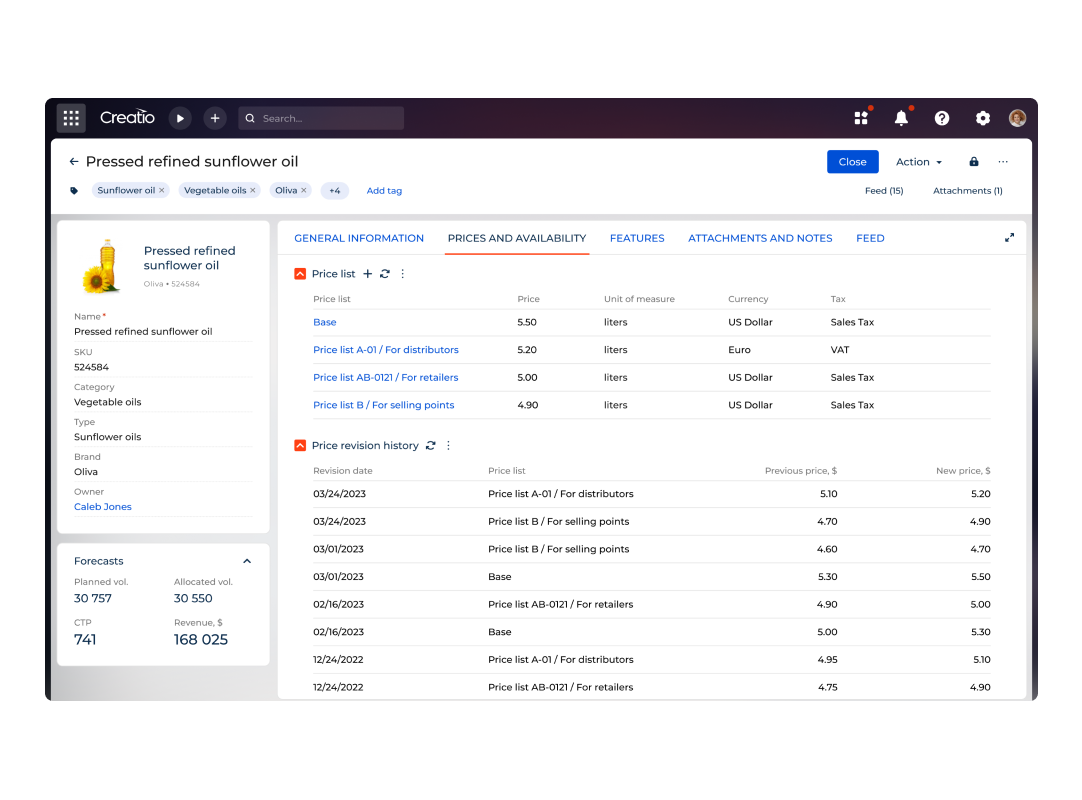
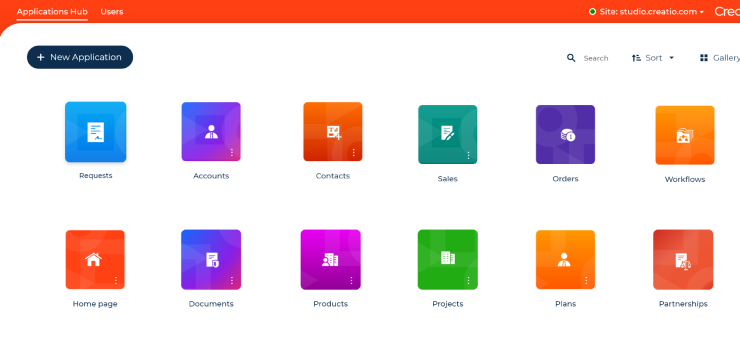
Predictive AI vs Generative AI
Feature | Predictive AI | Generative AI |
Goal | Forecasts future events and outcomes; provides valuable insights to support data-driven decision-making | Generates new, unique content |
How it works | Analyzes historical and real-time data using ML and statistical models to make predictions | Uses Large Language Models (LLMs) and deep learning to generate content based on patterns in analyzed data |
Business application | Data-driven decision making across all departments and industries | Content marketing, customer service automation, sales offers and follow-ups |
Key techniques | Regression models, decision trees, random forests, neural networks, linear and logistic regression | Transformer models (Chat GPT), Diffusion Models (DALL·E 3), Generative Adversarial Networks (GANs) |
Examples | Sales forecasting, credit scoring, audience segmentation, customer churn, stock levels optimization | Text and image generation, chatbots, code generation, music and video creation |
Human in the loop | Predictive AI continuously analyzes data. Humans are essential for making final decisions | Humans need to initiate content generation, edit and refine the output
|
Generative AI (GenAI) uses Large Language Models (LLMs) to create new content, such as text, images, code, and videos from descriptions in natural language. GenAI models learn statistical relationships within datasets and predict what should come next in any given sequence to generate output. Predictive AI, on the other hand, uses machine learning and statistical models to analyze and learn from vast amounts of historical and real-time data to predict future demand and sales, detect fraud attempts, recommend next best action to close a deal or resolve support case, and many more.
These two types of artificial intelligence can be used together to improve productivity, deliver better customer experiences, and increase revenue. For example, predictive AI can predict which products will be in demand next season, and generative AI can automatically generate personalized marketing content based on those predictions to capitalize on upcoming trends.
Predictive Analysis
Predictive analysis is an advanced form of data analytics that estimates the likelihood of specific events based on large volumes of historical data and current facts. It’s based on machine learning models, which identify correlations between different elements in selected datasets to predict values for any business object. Predictive analysis supports fast and accurate decision-making, frees users from routine tasks, and improves overall efficiency and productivity.