What Is Conversational AI? Definition, Benefits & Use Cases
Conversational artificial intelligence is a popular AI-powered technology designed to understand and imitate human interactions in a way that closely resembles real-life dialogs. Today, Conversational AI is provided via various solutions and tools — such as virtual assistants, chatbots, and AI agents — helping businesses to support customer service, internal operations, and employee productivity.
In this article, we will explore the fundamentals of conversational AI systems and the value they bring to organizations today.
What is Conversational AI?
Conversational AI is an advanced form of artificial intelligence that enables natural, human-like interactions through text, voice, or other communication means. It combines natural language processing (NLP), machine learning (ML), and foundational models — large-scale AI systems trained to handle various language tasks — to understand user intent, analyze context, and deliver relevant responses in a dialog format.
Unlike rule-based systems that follow predefined scripts, conversational AI continuously learns from every interaction. By analyzing user behavior, preferences, and past dialogs, it enables more personalized and efficient communications between people and software systems.
How Conversational AI Works?
Conversational AI relies on a combination of powerful technologies to better understand human language and deliver natural, accurate responses. Its large-scale language models and deep learning algorithms enable the system to generate context-aware outputs and improve over time by learning from vast datasets and advanced language patterns.
At the core of conversational AI is natural language processing (NLP) – an advanced capability that enables systems to analyze user input, recognize intent, and extract key information. NLP consists of natural language understanding (NLU) and natural language generation (NLG), which help an AI model to figure out what the user means and how to respond appropriately.
Dialog management is another essential component of conversational AI, acting as the orchestrator of the conversation. This system guides the AI through the entire interaction, including determining what happens next, what context to retain, when to ask follow-up questions, and how to guide the user toward a goal.
Finally, to enhance overall response quality and user experience, conversational AI applies advanced machine learning (ML) technologies that learn from historical interactions and adapt to user behavior. This allows the system to deepen the understanding of the user context and deliver more precise, human-like interactions at scale.
How Conversational AI Answers User Queries: A Step-by-Step Process Overview
To better understand how conversational AI systems work, here’s a simplified overview of a typical interaction between a human and an AI.
Step 1. Input Reception
The system receives a user query in any format, such as text, voice, or file-based data. For non-text inputs, the AI applies supporting technologies, such as automatic speech recognition (ASR), optical character recognition (OCR), and other solutions to interpret user inquiry.
Step 2. Input Processing & Analysis
Using advanced natural language processing and machine learning models, the system identifies the user's intent, extracts relevant details (such as names, dates, or keywords), and evaluates the conversational context to guide the response generation.
Step 3. Response Generation
Based on the analysis, the system generates an appropriate response. This may involve answering a question, retrieving data from connected systems, or performing a specific action. Natural language generation (NLG) ensures the output is clear, relevant, and conversational.
Step 4. Response Delivery
The system delivers an accurate response to user input in the form of a text message, voice reply, or other content. In many cases, conversational AI algorithms also use feedback from the interaction to fine-tune future responses through continuous learning.
Conversational AI vs. Generative AI: How Are They Different?
Conversational AI and generative AI (GenAI) are closely related technologies, but they serve different purposes and operate at different levels of abstraction.
- Conversational AI is designed specifically to simulate human-like interactions. Its main goal is to make information accessible through intuitive, human conversations. These systems do more than just generate responses — they interpret intent, track context, and maintain continuity across interactions to deliver accurate and coherent answers.
- Generative AI is a broader category of artificial intelligence focused on creating new content based on given prompts. In addition to conversations, generative artificial intelligence can also generate text, code, images, audio, and other content.
Earlier conversational AI systems were largely rule-based, following rigid scripts with limited flexibility. Today’s technologies, however, rely on modern generative AI models to engage in more dynamic, context-aware conversations, thus paving the way for a new generation of intelligent chatbots and AI agents.
Key Benefits of Conversational AI Technologies
Modern conversational AI applications offer various advantages beyond basic chat functionality, including:
- Improved Customer Experience. Conversational AI enables businesses to deliver accurate, seamless, and personalized support across every stage of the customer journey. By understanding intent, maintaining context, and generating human-like responses in real time, the AI system creates more natural digital experiences that drive stronger customer engagement and satisfaction.
- Enhanced Operational Performance. Conversational AI reduces the friction of navigating complex systems by allowing users to access information via inquiries in natural language. This enables businesses to increase day-to-day productivity and minimize human error.
- Cost Efficiency. Conversational AI lowers operational costs by automating high-volume tasks such as support inquiries, task routing, or data entry. With less manual workload and smarter task distribution, businesses can cut operational expenses while maintaining high levels of performance and quality.
- Improved Scalability. Conversational AI models can handle thousands of simultaneous interactions across multiple channels, time zones, and languages. This enables organizations to scale their customer support elastically during peak times or when growing their customer base.
- Enhanced Employee Productivity. Conversational AI supports internal teams by acting as a self-service assistant across various departments, such as HR, IT, sales, and others. By minimizing time spent on repetitive tasks or information searches, employees can focus on higher-value work and complete tasks faster.
Common Examples of Conversational AI Systems
LLM-Powered Chatbots
LLM-powered chatbots represent the latest evolution of advanced conversational AI technologies. Unlike traditional script-based bots, these intelligent systems generate dynamic, context-aware responses using advanced AI models such as GPT, Claude, and Gemini.
Today, LLM-powered conversational bots are used across customer support platforms, self-service portals, internal knowledge assistants, and various enterprise tools. By making digital communication faster, more accurate, and intuitive, these AI-powered chatbots enable businesses to improve operational performance and build stronger customer relationships.
Voice Assistants
Voice assistants combine speech recognition and conversational artificial intelligence to provide hands-free, natural communication between users and digital systems. With real-time speech-to-text capabilities, voice assistants allow users to retrieve information, perform tasks, and access services through simple verbal commands.
In the business landscape, voice assistants are becoming essential tools for streamlining operations and improving productivity in voice-first environments. In healthcare, they help manage patient records more efficiently. In logistics, they support warehouse teams in tracking inventory without interrupting tasks. Banks, Telcos, and retailers are also adopting voice-driven solutions, with enterprise-ready platforms like Amazon Alexa for Business and Google Assistant integrations becoming more common.
Conversational AI Agents
Conversational AI agents are intelligent, context-aware assistants that are about to become a core component of modern digital applications and workflows. These solutions go beyond answering questions, helping users streamline and automate entire end-to-end processes through intuitive, dynamic interaction.
Powered by advanced NLP models and machine learning algorithms, AI agents can support and augment human decision-making across a wide range of tasks — from summarizing documents and suggesting next steps to independently executing multi-step processes based on conversational instructions. For instance, virtual AI assistants used in CRM software can autonomously access leads and opportunities, execute marketing campaigns, process service requests, and provide insights on how to best resolve a specific case.
Domain-Specific Virtual Assistants
These conversational AI assistants are tailored to specific industries or business functions and enable expert-level support using natural, human language. They can be deployed internally — to assist employees with knowledge access, compliance, or workflow guidance – or externally to deliver seamless, intelligent customer interactions.
The results speak for themselves: Erica, Bank of America’s virtual assistant, now handles over two million interactions per day, helping users manage everyday financial needs through conversational guidance. Similar assistants are now used across industries, enabling businesses to improve service quality, automate interactions, and create more meaningful, customer-centric experiences.
Use Cases: Where Conversational AI is Making an Impact
Driven by the need for intelligent automation, real-time engagement, and scalable support, the conversational AI market is introducing new ways to enhance both customer-facing and enterprise workflows.
Here are five high-impact use case categories that show how conversational AI is transforming operations across different industries:
1. Information Retrieval & Guidance
Conversational AI enables users to quickly access relevant information and receive real-time guidance through natural, dialog-based interactions.
- Finance & Banking: Conversational AI can guide customers through comparing account options, understanding loan terms, checking real-time balances, and opening new accounts — without the need for human intervention.
- Telecommunications: AI chatbots and voice assistants help users explore plan features, evaluate upgrade options, or retrieve device-specific support content through intuitive conversations.
- Retail: Conversational AI can answer questions throughout the buying cycle, providing on-demand information on product specifications, stock availability, return policy details, and loyalty program information.
- Healthcare: AI-driven assistants can support patients by identifying appropriate specialists, explaining symptoms in user-friendly language, verifying insurance coverage, and confirming appointment times in real-time.
2. Workflow Automation
Conversational AI solutions streamline and automate the execution of various processes with ease, eliminating the need for human involvement.
- HR & Internal Operations: Employees can effortlessly update personal data, submit vacation or sick leave requests, complete onboarding checklists, or access policy documentation through self-service AI chatbots.
- Logistics: Conversational AI helps logistics teams assign deliveries, update shipment statuses, or check fleet availability by executing tasks directly from integrated backend chatbot systems.
- Manufacturing: AI technologies assist in initiating maintenance requests, reporting incidents, or reordering parts by recognizing task triggers through operational conversations.
3. Transaction Support
AI-powered conversational tools can handle complex transaction requests, by securely guiding users through account-related actions, reducing friction, and ensuring accuracy.
- Banking: AI assistants process bill payments, fund transfers, card locking, and recurring payment setup.
- Insurance: Policyholders use AI chatbots to initiate claims, modify payment methods, update coverage details, or receive billing assistance without navigating multiple tools.
- Retail & eCommerce: Conversational AI enables shoppers to complete purchases, apply discounts, update delivery information, or cancel orders within a single interaction.
4. Personalized Recommendations
Conversational AI applies a data-driven approach to deliver a personalized user experience. By continuously analyzing user behavior, preferences, and past interactions, these solutions provide the right suggestions that align with individual goals and needs.
- Real Estate: Virtual human agents match prospective buyers or tenants with property listings that fit their preferences, budget, and lifestyle needs, yet can refine results as the conversation progresses.
- Financial Services: Conversational AI recommends investment options, credit products, or budgeting tools tailored to a customer’s financial goals, spending history, and risk profile.
- Manufacturing: Conversational AI assists operators or engineers by recommending maintenance procedures, parts replacements, or workflow adjustments based on equipment status, usage data, and production goals.
- Logistics & Supply Chain: AI helps logistics coordinators choose optimal delivery routes, adjust schedules, or prioritize shipments based on real-time inventory, order urgency, or delivery constraints.
5. Scalable, Multi-Step Conversations
Conversational AI models can effectively handle thousands of conversations in parallel, retaining context across sessions and delivering consistent responses to its users. These capabilities are essential in processes where users need guidance through multiple stages or where decisions depend on previous responses.
- Public Sector & Government Services: AI assistants help citizens complete multi-step applications, check eligibility for programs, or schedule services, ensuring consistency and clarity throughout the interaction.
- IT & Technical Support: Virtual agents guide users through step-by-step troubleshooting, adjust instructions based on user feedback, and escalate cases only when necessary. They can also preserve the full interaction history for human agents, which helps to enhance service efficiency and customer satisfaction.
- Retail: Conversational AI supports shoppers through guided product selection, checkout, and post-purchase support—retaining context across sessions to offer a seamless, personalized shopping experience.
Key Challenges of Conversational AI Technologies
Despite rapid AI adoption in every line of business, it is important to acknowledge the inherent challenges for conversational AI, such as:
- Accuracy & Context Understanding. Conversational AI models may struggle to interpret user intent, especially in knowledge-specific interactions. To keep the responses relevant across longer conversations, AI vendors must fine-tune their models, incorporate domain knowledge, and improve context retention mechanisms, such as session memory and intent tracking.
- User Trust. Concerns around data privacy, transparency, and reliability often prevent users from fully relying on AI systems, especially when dealing with sensitive or mission-critical queries.
- Bias in AI Models. Conversational AI learns from the data they are trained on, which means they can unintentionally reflect and reinforce biases related to gender, race, or culture. This raises ethical concerns in various regulated industries and requires proactive monitoring, diverse training data, and a long-term commitment to responsible AI development.
- Privacy & Security Risks. Conversational AI operates with large volumes of personal and sensitive data, which makes it potentially vulnerable to misuse or breaches. That is why AI systems must consistently improve data encryption, access controls, and compliance with privacy regulations (like GDPR or HIPAA).
- Adoption Issues. Despite growing interest in conversational AI, many organizations still face challenges in its adoption, ranging from integration with legacy systems to insufficient technical expertise and uncertainty around ROI. According to Deloitte’s recent research, only 26% of organizations consider themselves “seasoned” in AI adoption — a gap that can only be closed through greater awareness, clear business strategies, and stronger implementation frameworks.
As conversational AI continues to mature, most of these challenges will be addressed through improved model performance, responsible development practices, and increased awareness of AI integration and its strategic business value.
The Future Trends of Conversational AI Tools
Driven by the growing demand for intelligent automation, real-time engagement, and scalable support, conversational AI continues to gain momentum across industries.
According to Grand View Research, the market is expected to grow from $14.29 billion in 2025 to $41.39 billion by 2030, unlocking new, innovative use cases across both customer-facing and internal business workflows. Below, we have reviewed the key trends that will shape the future of conversational AI.
AI Personalization & Humanation
The next generation of conversational AI tools will go beyond surface-level personalization to deliver interactions that feel natural, intuitive, and user-friendly. These systems will require less input to understand intent, drawing on past interactions, preferences, and behavioral cues to generate faster and more accurate responses.
By adjusting tone, phrasing, and dialog flow to match individual communication styles, AI assistants will create conversations that feel far less robotic and more human-like. As a result, this approach enables businesses to boost customer satisfaction, increase engagement, reduce friction, and improve the overall effectiveness of digital service and support channels.
Multimodality
Earlier AI systems were limited in scope, often processing only a single type of input, such as text or images. Multimodal AI systems, however, will be able to understand and respond using a combination of output formats, including text, voice, images, video, and other types of data — all within a single interaction. This gives users the freedom to communicate more naturally, whether it’s speaking, typing, or sharing visual input.
Multimodality is becoming a defining trend in conversational AI, as it opens the door to richer, more intuitive experiences across channels and devices. While current AI systems are still learning how to accurately match user intent and provide comprehensive responses, the future of conversational AI will seamlessly blend multiple content types, such as annotated visuals, synthesized speech, and layered text. According to Gartner, 40% of all generative AI outputs will be multimodal by 2027, signaling a major shift in how AI supports and enhances user interaction.
Emotional Intelligence
Emotional intelligence will become a defining capability of next-generation conversational AI, making interactions more meaningful and human-like. By analyzing sentiment, tone, and language patterns in real-time, these systems can recognize user emotions and context to adjust AI responses with empathy and an appropriate tone.
For businesses, emotionally intelligent AI helps build trust and enhance service quality in cases where clear, compassionate communication is essential. This is especially valuable in industries that depend on customer interactions, such as healthcare, financial services, and telecom.
Creatio.ai: Empower Your Business with Conversational AI Technologies
Creatio offers one platform to automate industry workflows and CRM with AI, no code, and a maximum degree of freedom. Unlike traditional platforms that offer AI as an expensive add-on, Creatio embeds predictive, generative, and agentic AI directly into business workflows at no extra cost.
With Creatio AI, companies can use ready-set-go industry solutions or build custom AI-native applications tailored to their unique business needs. The platform is powered by role-based AI agents, which support a wide range of use cases, such as content generation, decision-making, process optimization, and many more. By embedding intelligence directly into daily operations, Creatio AI enables teams to collaborate more effectively, act on insights faster, and drive results across the entire workflow cycle.
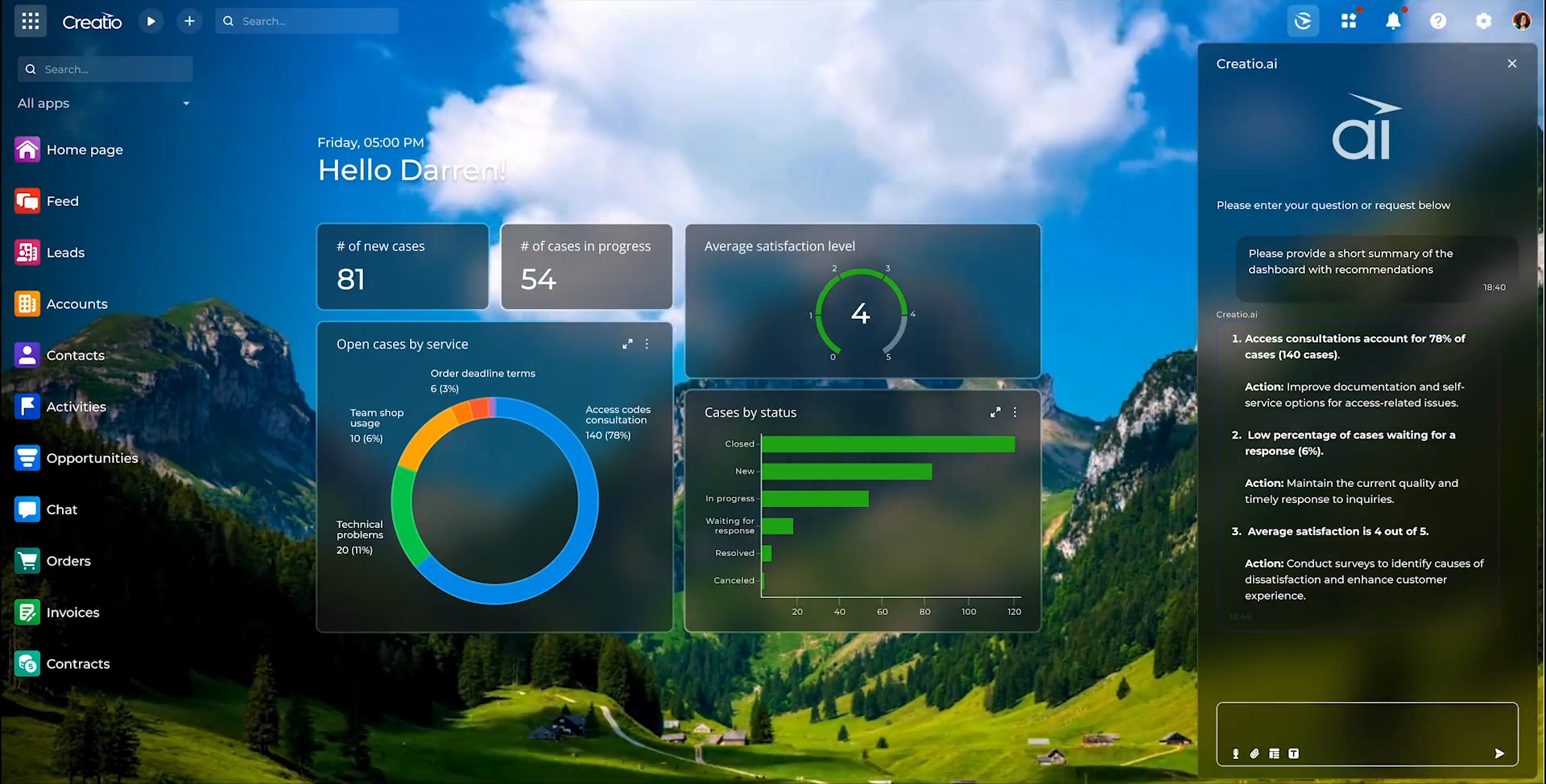
Sales teams use Creatio AI to streamline and enhance every stage of the customer conversion cycle through a single platform. Creatio AI can generate personalized messages, identify upsell and cross-sell opportunities, summarize meetings, and provide real-time insights into pipeline health and deal progress. This helps sales reps prioritize the right opportunities, improve forecasting accuracy, and stay focused on closing more deals.
Marketing teams rely on Creatio AI to accelerate campaign planning and execution through simple, natural language prompts. Creatio AI can support audience analysis, automate content generation, and recommend campaign improvements to maximize the marketing ROI.
Customer service teams use Creatio AI to deliver faster, more accurate support through intelligent, real-time assistance. Creatio AI can summarize incoming cases, suggest the next best steps, personalize and automate responses, and analyze customer sentiment with ease. By learning from each interaction, Creatio AI also helps automatically expand the knowledge base with new articles and updates.
Unlike rigid traditional systems with fragmented AI add-ons, Creatio provides a unified AI-native platform with end-to-end automation for business workflows. Its role-based AI agents enhance visibility, forecasting, and execution across various departments, enabling teams to collaborate seamlessly and drive consistent results throughout the operational cycle.
Finally, Creatio AI gives businesses full control over automation with intuitive, no-code tools. With drag-and-drop interfaces, customizable workflows, and embedded intelligence, businesses can accelerate execution, improve forecasting accuracy, and unlock new opportunities for growth.
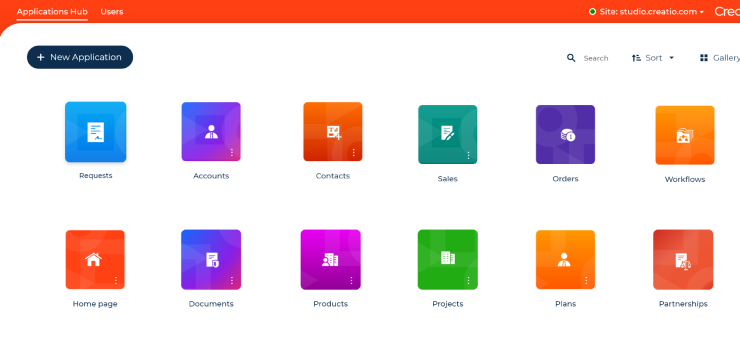