What is Artificial Intelligence (AI) in Finance? The Future of the Financial World
Artificial Intelligence is transforming the financial services industry by enhancing customer convenience, boosting business productivity through automation, and handling complex operations like processing vast amounts of data and identifying trends that would be impossible for humans.
However, the integration of AI in finance comes with its own set of challenges and cautions. Businesses need to address issues such as selecting the right software and ensuring compliance with regulatory and ethical standards.
In this article, we will explore the reasons for implementing AI in financial services, delve into common use cases for AI in finance, examine the current challenges faced by financial institutions, and discuss emerging trends that will shape the future of AI in finance.
What is AI in Finance?
Artificial intelligence in finance refers to a suite of advanced technologies designed to replicate human intelligence and decision-making processes. These technologies enable financial institutions to analyze complex data, automate operations, make informed investment decisions, and enhance security measures to protect financial activities.
AI technologies that are utilized in financial sector include:
- Machine learning (ML) allows systems to learn and improve autonomously using neural networks. By feeding large amounts of data into ML algorithms, financial institutions can train models to solve specific problems and gain insights for ongoing improvement.
- Deep learning (DL) is a specialized ML technique using multi-layered neural networks, allowing systems to learn from vast datasets like financial transaction histories. DL is used in credit scoring, algorithmic trading, chatbots, and anti-money laundering.
- Natural language processing (NLP) centers on understanding human language. In finance, NLP analyzes customer conversations, news, regulatory documents, internal communication such as meeting transcripts and other texts to support process automation and discover data-based insights.
- Computer vision (CV) allows computers to interpret and understand digital images and videos. In finance, CV is used for tasks like processing checks, monitoring physical assets, analyzing facial expressions, and more to combat fraud and gather insights.
By leveraging AI, the finance sector can optimize performance, increase its productivity levels, reduce risks, and deliver customer experience that meets evolving market demands.
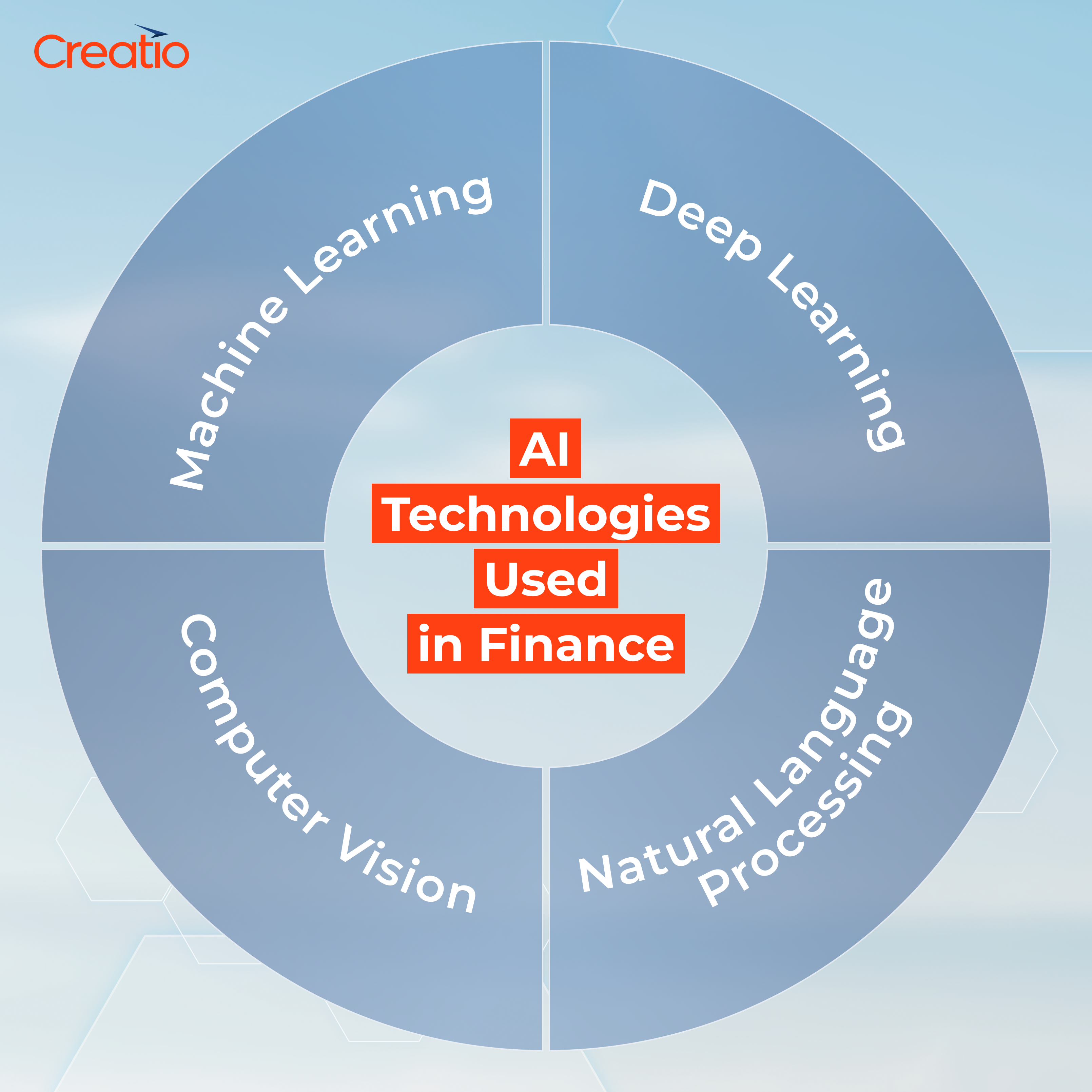
How AI is Used in Financial Services
Currently, AI's core functionalities in financial organizations include processing and analyzing data to quickly gather insights, identifying trends and deviations from these trends, and automating workflows and communication. These applications can lead to significant business benefits.
AI algorithms can automate manual tasks, allowing employees to focus on higher-value work. For example, AI chatbots now handle routine customer service queries about account balances and payments, freeing up employees for more complex tasks.
Automating information-gathering and analysis supports KYC and customer due diligence processes, helping finance professionals verify customers and transactions, and enhancing fraud prevention and detection. Machine learning models can analyze millions of transactions to detect subtle patterns indicating fraud more quickly and accurately than humans.
Additionally, by analyzing customer data and automating conversations, AI agents can provide personalized product recommendations based on transaction history and spending patterns. This not only improves the customer experience but also positively affects conversion rates for companies.
Overall, AI in finance supports the following activities:
- Personalization
- Risk management
- Fraud detection
- Regulatory compliance
- Customer due diligence
- Front-office support
- Forecasting and predictive modeling
- Business intelligence
Benefits of AI in Finance
Enhancing operational excellence
AI streamlines operations for financial organizations by helping them avoid errors that can easily be made by human workers due to the volume of data and operations. Routine processes, such as data entry, transaction processing, and compliance checks, are prone to mistakes when performed manually. AI systems, however, can execute these tasks with high precision and consistency, ensuring accuracy and reliability.
With its ability to process vast data sets, artificial intelligence improves the accuracy of forecasting, planning, and modeling. For instance, in sales forecasting, AI algorithms can analyze vast amounts of data and identify patterns that humans might miss. This leads to more accurate predictions and better decision-making.
Better customer experience
AI enhances the way finance professionals engage with clients and automates routine customer interactions, making services more accessible. For instance, customers can apply for loans and credit cards and receive immediate responses from an AI chat bot without waiting for an available agent. The same AI bot can flag more complicated cases that require human intervention, ensuring that all customers receive timely and appropriate attention, leading to higher overall satisfaction.
Additionally, AI enables finance professionals to provide more personalized interactions. Marketing departments can use AI-driven data to tailor their campaigns, while sales representatives and customer service agents can offer customized communication and experiences.
For example, in wealth management, an AI assistant can compile data from various systems to prepare comprehensive summaries for client presentations, supporting advisors with accurate and timely information. AI-powered automation can also offer personalized recommendations for customers, creating opportunities for upselling.
Increased productivity
AI increases the productivity of financial organizations by automating time-consuming tasks, such as market research or fraud detection, which reduces the workload on employees and minimizes errors.
Moreover, automated tasks are performed at a higher velocity, meaning a higher volume of operations with fewer resources engaged. For instance, AI-driven algorithms can execute trades at high speed and with precision, optimizing trading strategies and increasing returns while reducing the need for manual intervention.
Improved decision-making supported by data insights
AI supports decision-making in financial organizations by unlocking deeper insights from both structured and unstructured data. It reveals patterns and trends that would be impossible for humans to detect alone. This advanced data analytics enables financial institutions to make more informed, strategic decisions, ultimately improving their performance.
For example, banks can utilize AI algorithms to swiftly analyze market data and news to improve risk management and guide investment decisions and trading strategies.
Improved security
AI systems and machine learning models can spot potential threats more effectively than human analysts due to the real-time calculations and analysis of transactions. By closely monitoring purchase behavior and comparing it to historical data, AI can flag anomalous activity, automatically alert both institution and customer to verify the purchase or transfer in real time, and if needed, take action to resolve it.
AI in Finance: Common Use Cases
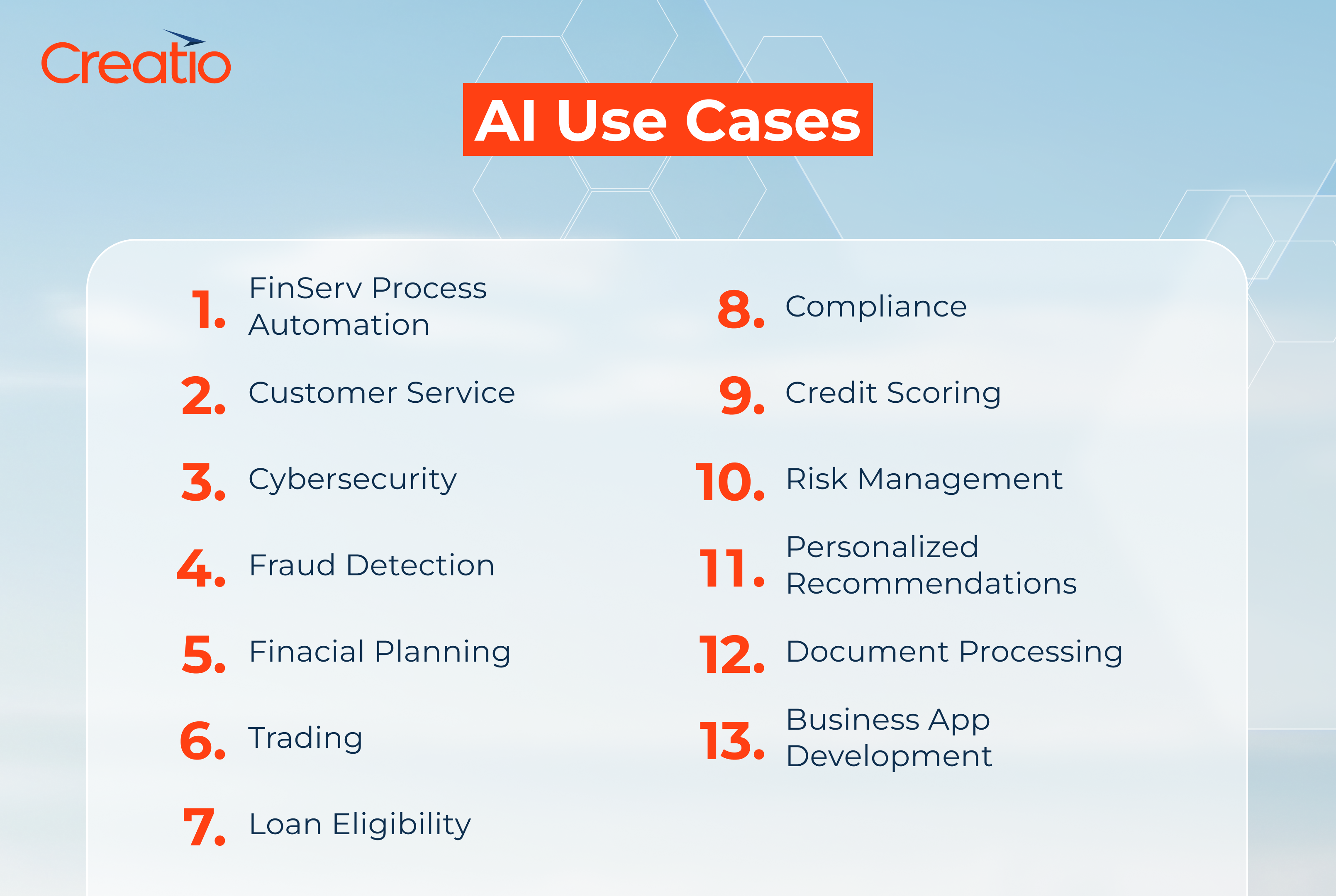
The applications of AI tools in the financial industry are constantly expanding and evolving along with the technology itself. Here's a list of current use cases for AI that can bring business value to any financial institution.
- Automating traditionally manual banking processes. AI can automate repetitive and time-consuming tasks, allowing financial institutions to process large amounts of data faster and more accurately. For example, ERP systems with built-in AI technology can now scan physical invoices, identify the key information such as supplier name, materials purchased, and associated cost, and enter it into their ERP systems automatically. Oracle uses their AI solutions for accounting to streamline their regular financial reports, which frees up their financial team to perform tasks that require more focus.
- Customer service. Conversational AI chatbots enable banking customers to access account information quickly and efficiently around the clock. These technologies reduce the need for human intervention, deliver personalized customer service and recommendations and enhance fraud protection and cybersecurity for consumers. You could work with an AI assistant to submit your issues and track its progress without waiting for a human employee to respond during the working hours of the business. For instance, American Express offers a chatbot called Ask Amex to dispute charges. AI agents help resolve flagged legitimate purchases without waiting on hold or dealing with confusing websites. Customers can give the details of the flagged transaction to the AI agent that can help customers explain the transaction, upload receipts, and verify their identity.
- Cybersecurity. With AI, you can automate cybersecurity by continuously monitoring and analyzing activity and network traffic to detect, prevent, and respond to cyberattacks and threats.
- Fraud detection and prevention. Thanks to ML models and deep learning, AI can analyze customers’ buying behavior and trigger alerts when unusual patterns, such as fraudulent transactions, financial crime, or spoofing in trading, are identified. This enhances security in trading, online banking, and credit card transactions.
- Financial planning for customers. AI can help people manage their personal finances by analyzing goals, spending patterns and risk tolerance to develop budgeting advice and savings strategies. AI agents utilize sophisticated algorithms to provide affordable, personalized investment advice based on customers’ goals, risk tolerance and market conditions. For example, an AI research assistant can help identify reliable opportunities and recommend how to capitalize on them, while a financial coach powered by generative AI can help you make smarter decisions with your spending.
- Trading. AI can be utilized in trading algorithms that analyze market trends and historical data, enabling faster decision-making and trade execution than human capabilities.
- Loan eligibility. Lenders are using AI to quickly analyze data and determine customers' creditworthiness. By automating risk assessment, credit scoring, and document verification, AI improves the prediction and evaluation of loan risks, streamlining the approval process for borrowers.
- Compliance. AI can track and automate monitoring and reporting processes to ensure compliance with local and international regulations.
- Credit scoring. AI can analyze diverse data beyond financial records, such as social media activity and online behavior, to extract insights and assess customers' reliability and credit eligibility.
- Risk management. AI can analyze vast datasets to assist financial organizations in accurately assessing and mitigating risks, thereby enhancing security and stability in the financial sector.
- Personalized marketing communication and recommendations. AI can provide personalized recommendations for financial products and services, such as investment advice or banking offers tailored to customer journeys, peer interactions, risk preferences, and financial goals. Moreover, by analyzing customer journeys, generative AI can help create targeted emails and online campaigns that lead to higher conversions and better business outcomes.
- Document processing. AI can extract and analyze data from documents to streamline document-intensive processes like loan servicing and investment opportunity discovery. This report provided by Forrester describes an insurance company that uses a text analytic agent to rename, file, and categorize documents. According to the firm’s owners: “With AI agents, we were able to remove 25% of responsibility from assistants, allowing them to focus on more meaningful tasks rather than mundane work. Tasks like renaming documents are time-wasting activities that crush everyone’s spirit.”
- Business app development with generative AI. The combination of no-code tools and generative AI allows financial services organizations to develop their own business apps to further strengthen their digital transformation. For example, Creatio Copilot lets you build apps to automate unique business processes based on natural language prompts.
Challenges in Financial AI Implementation
While AI technology can bring incredible business value to financial services organizations and business leaders, there are still some challenges. In this section, we wanted to go through the challenges posed by AI tools and give tips on how to resolve them.
Flaws in communication
Despite the many benefits of conversational AI, customers of financial services companies may perceive it negatively due to imperfections in non-human communication. AI chatbots can come across as too impersonal or formulaic, and their capability to resolve complex issues is limited, leading to frustration and reduced engagement.
To combat these issues, it is crucial to carefully craft and implement sophisticated technology that can handle complex queries and provide personalized responses. Ensure that your bots are capable of understanding and appropriately responding to a wide range of customer interactions and will transfer the conversation to a human agent at the first moment of miscommunication and frustration.
Additionally, regularly updating AI algorithms to better mimic human-like interactions and monitoring customer feedback can help maintain high customer satisfaction.
The need for outstanding development resources
Companies often site development and logistical problems when talking about the failures to launch their AI projects. Developing bespoke AI technology often requires a lot of talented software engineers, making these tools inaccessible for some organizations.
Companies should prioritize smart resource allocation, choose effective business automation software, and leverage no-code tools to mitigate this issue. Ensure that skilled professionals are directed toward high-impact areas. Selecting robust business automation platforms with powerful turn-key AI tools accelerates the implementation process.
No-code development platforms, like Creatio, enable institutions to create their own ML models without requiring extensive coding expertise. This approach not only reduces dependency on specialized developers but also accelerates project timelines and enhances flexibility, ultimately improving the chances of successful AI project deployment.
IT and data infrastructure required for AI
Managing AI workloads with massive data volumes and intensive model training can be prohibitively expensive due to the server power required. Although many institutions opt for cloud infrastructure, stringent regulatory requirements around data security and residency often pose barriers to cloud adoption.
The volume of unstructured data in organizations is another challenge. Clean, representative data for training AI models is essential, as the quality of these models directly depends on the quality of data used. For AI solutions to function effectively, data must be organized in an orderly fashion.
However, many banks have fragmented data architectures spanning decades-old systems. Additionally, integrating modern AI tools with these legacy IT systems can be challenging.
It's recommended to implement robust data management processes to ensure data quality and integrity. You can also utilize customizable business tools that facilitate data governance and the integration of AI with your system.
Security and compliance
Financial organizations handle sensitive personal information daily thus security is a huge priority. AI solutions must protect this data and adhere to industry- and region-specific regulations, from credit decisions to trade surveillance. This compliance often involves extensive record-keeping and model documentation.
Ensure AI systems you use have robust security measures to protect sensitive information and are designed to meet specific industry and regional guidelines. Additionally, review the governance capabilities provided by the platforms you use and establish proactive governance procedures.
AI ethics
AI, trained on human-provided data, can inadvertently pick up and incorporate biases into its models, potentially leading to discriminatory decisions. For instance, if historical data around credit scores reflects biases against certain demographic groups, an AI model trained on this data might continue to disadvantage these groups.
To combat this issue, financial institutions should establish robust oversight and clear rules for AI application and implement these strict ethical guidelines.
How Creatio's AI-Powered No-Code Platform Revolutionizes FinServ
Creatio is a no-code platform for automating workflows and CRM that heavily incorporates AI, including a multipurpose AI assistant Creatio Copilot. With its no-code capabilities, intuitive interface, and composable architecture, Creatio empowers financial organizations to adapt and customize workflows without the need for software developers.
Additionally, Creatio offers several vertical solutions for various financial industries, including banking, credit unions, and insurance, which provide plenty of turn-key capabilities to help automate financial processes.
As for the AI features, Creatio offers robust out-of-the-box capabilities, including:
- Prepackaged AI and ML models for data analysis, intelligent scoring and predictions, customer segmentation, case routing, and more.
- AI-driven business rules to automate routine tasks across marketing, sales, and customer service.
- AI-powered no-code tools for AI-assisted development, enabling users to create and customize solutions easily.
In addition to that, you can create your own ML models and business rules to automate specific workflows.
Creatio Copilot integrates generative AI and no-code technology, enabling users to leverage pre-built AI scenarios and create new ones via intuitive no-code interfaces. It is a dedicated workspace for developing and customizing AI models and use cases based on natural language descriptions.
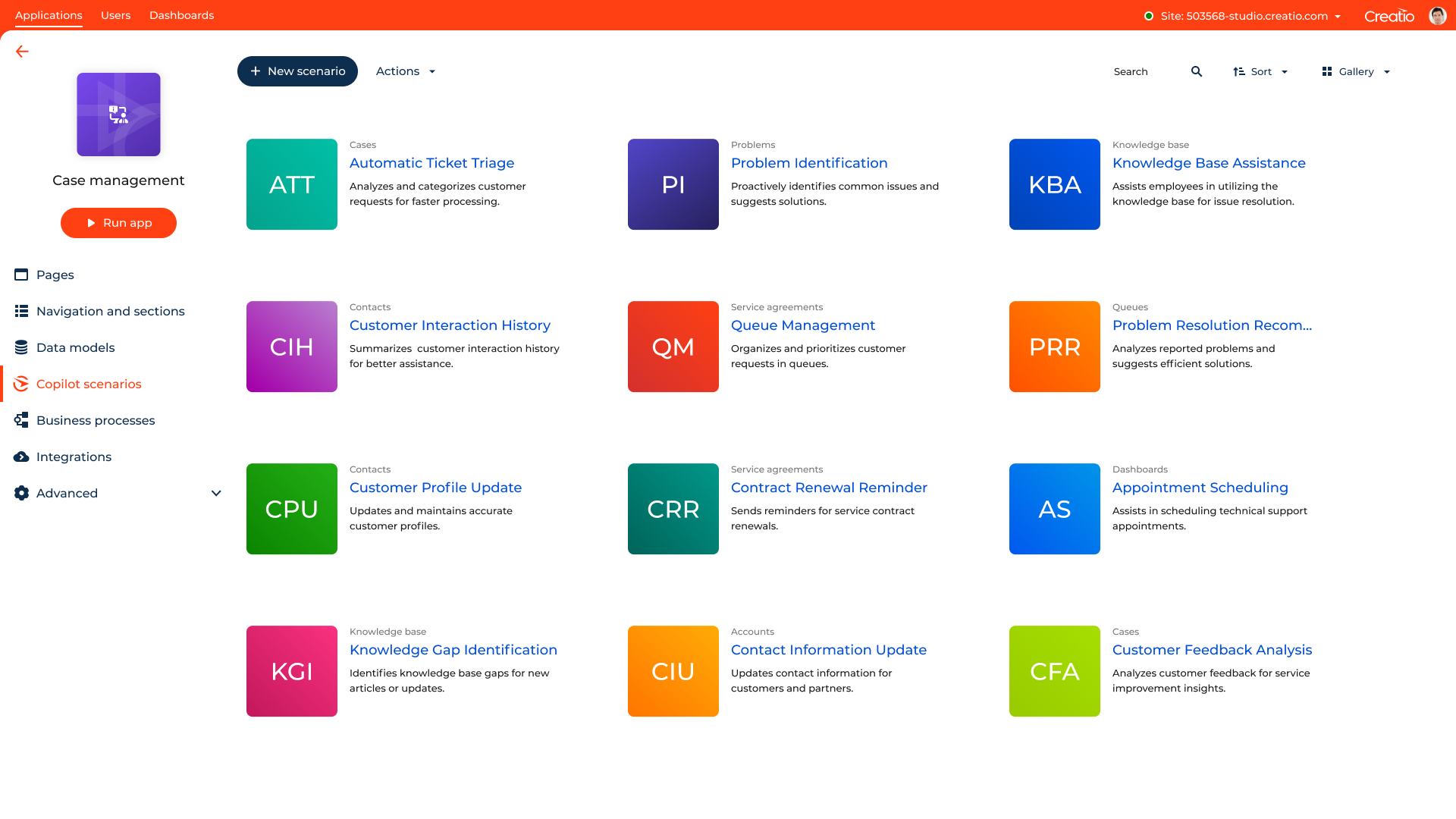
Some use cases for Creatio Copilot
For end users, Creatio Copilot acts as a virtual assistant, fetching specific information like a quick summary of a customer's financial history, and creating bespoke dashboards with visualizations, charts, projections, and analyses on demand.
Sales professionals can use Creatio Copilot for intelligent customer scoring, interaction summaries, and real-time coaching tips. Marketers can design optimal campaign flows and target responsive audiences. Customer service agents can automate case analysis and deliver personalized responses effortlessly.
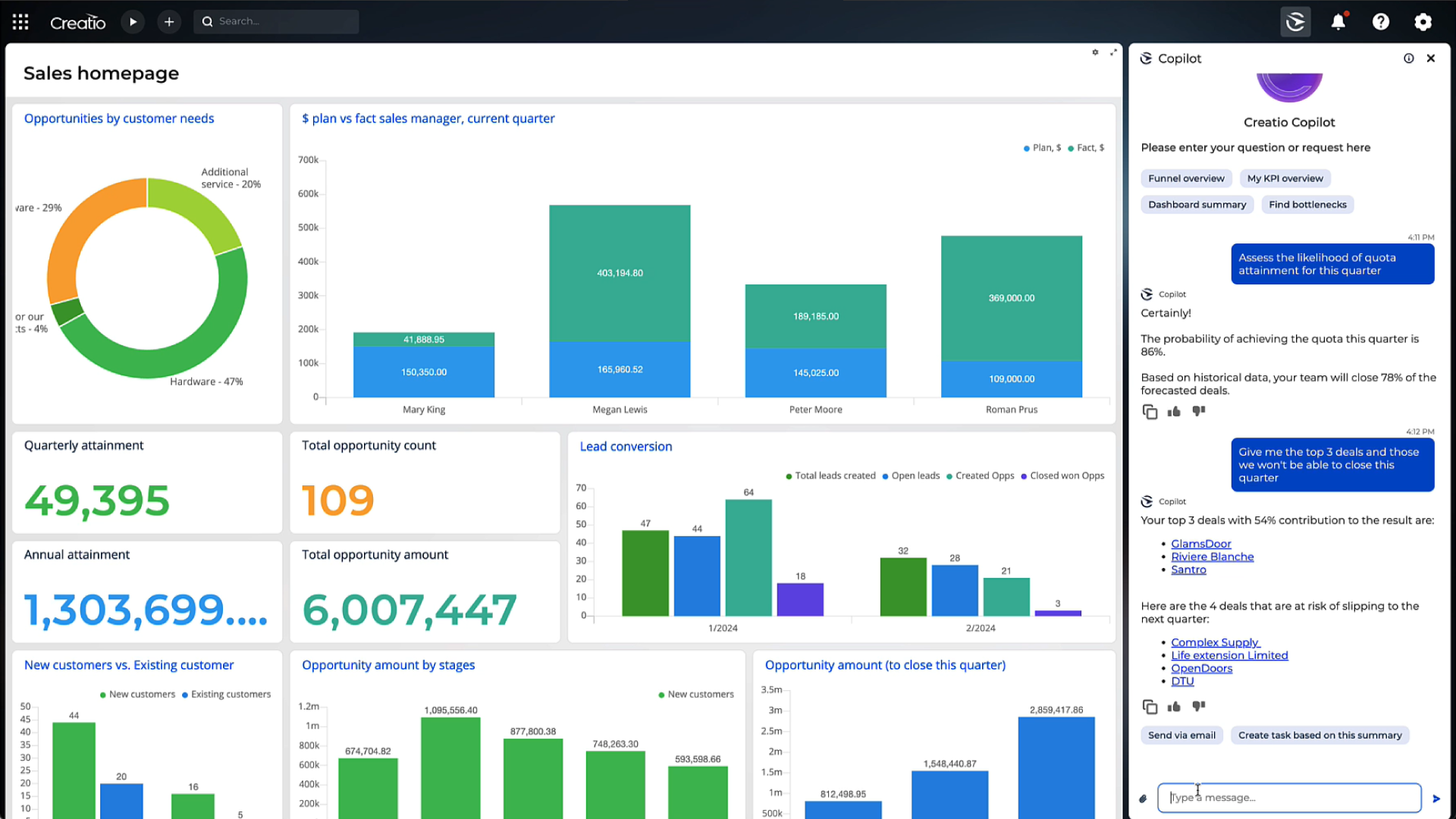
Creatio Copilot assistant summarizing sales reports
Moreover, by leveraging AI for predictive analytics and actionable insights, Creatio equips business leaders to make informed decisions and drive revenue growth effectively.
Sign up for Creatio's free trial and try AI automation with a maximum degree of freedom!
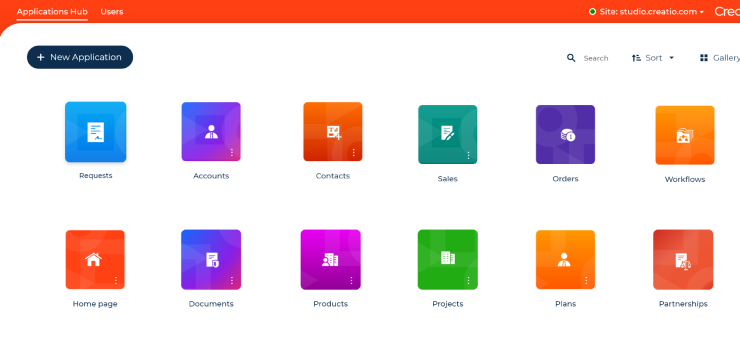
The Future of AI in Financial Services
AI is bound to gain widespread application in data analysis, identifying patterns, automating processes, and making personalized recommendations. The latter will be especially prominent with finance companies aiming to implement personalization at scale. As for customer service, AI-powered chatbots and digital wallet solutions will become more advanced and be able to mimic human intelligence at an impressive level.
In investments and trading, AI is poised to make accurate market predictions and execute sophisticated investment strategies. However, proper governance is essential as AI assumes a greater role in financial decision-making.
Machine learning will transform financial risk assessment and regulation by analyzing alternative data and detecting risks that humans might miss. AI will assist regulators, but transparency and accountability remain crucial.
All in all, the future of AI in finance lies in hybrid intelligence systems that integrate AI with human expertise, ethics, and emotions, ensuring a balanced and ethical approach to financial decision-making.
In Conclusion
AI in the financial sector is used to analyze data, automate operations, manage risk, and make better decisions. By embracing AI responsibly, organizations can unlock new opportunities for customer satisfaction and growth.
Experience the robust governance capabilities and limitless customization options of Creatio's no-code platform and discover the power of no-code and AI together.