AI in Banking - How Artificial Intelligence Can Be Used in Banking Industry
Artificial Intelligence significantly impacts the banking sector, bringing between 200 to 340 billion USD of added value and growing industry profits by up to 15 percent (Statista). It helps businesses drive innovation, enhance competitiveness, and secure a leading position in the finance market.
According to The Economist, over 80% of senior banking executives believe adopting AI is the key to success in the financial industry.
As AI technology continues to advance, it increasingly influences how banks operate, improving efficiency, security, and customer experiences. This article explores the role of AI in banking, including its use cases, implementation strategies, and potential risks.
What is AI in Banking?
Artificial Intelligence in banking refers to using advanced algorithms and machine learning techniques to enhance internal operations and customer-facing applications in the finance industry. AI technology helps automate and optimize processes such as customer service, fraud prevention, risk and investment management, and personalized financial advice.
Multiple AI-powered tools are influencing the operational efficiency of the banking industry. Chatbots and virtual assistants, powered by AI, provide 24/7 customer support, handling simple inquiries and transactions seamlessly. Meanwhile, machine learning models analyze large volumes of data to identify suspicious activities and prevent fraud. Additionally, AI-driven analytics offer personalized financial insights and recommendations to clients, further enhancing the customer experience.
According to Statista, the finance sector has one of the highest Artificial Intelligence adoption rates across all industries. By leveraging AI, banks can improve productivity, reduce costs, provide better, more secure services to their clients, and much more.
Artificial Intelligence Use Cases in Banking and Finance
AI technology has the potential to boost the banking and finance sectors by introducing innovative solutions that improve efficiency, security, and customer satisfaction.
These are some of the key use cases of AI in banking and finance:
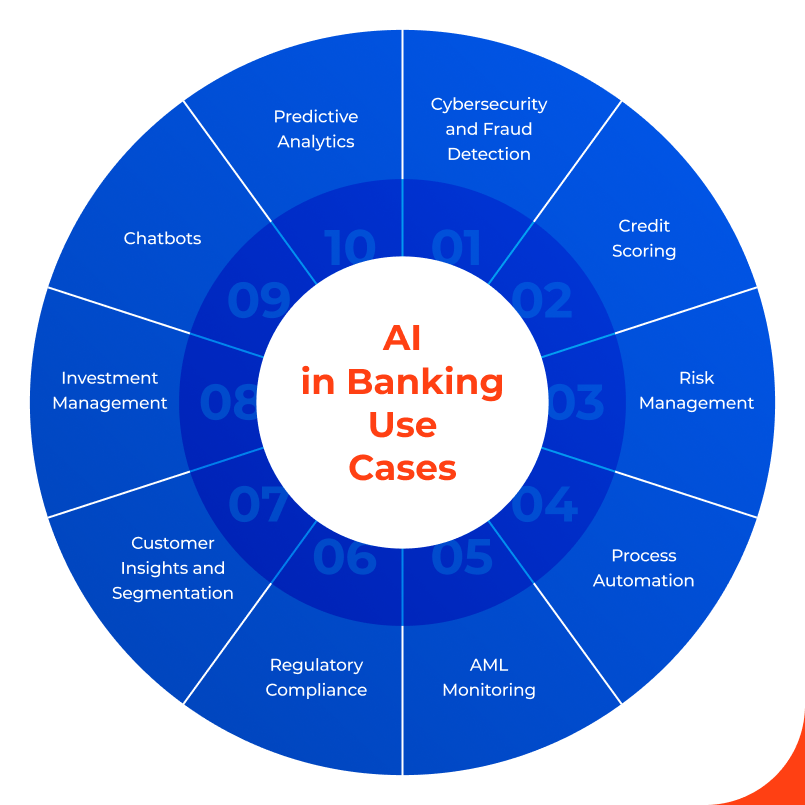
Cybersecurity and fraud detection
AI plays a critical role in enhancing cybersecurity and fraud detection within the banking sector. AI-powered algorithms can detect unusual patterns and anomalies in real time, identifying potential threats much sooner than humans.
AI systems analyze vast amounts of data to recognize malware and phishing attacks, adapting continuously to evolving cyber threats. By automating threat detection and response, AI helps banks protect sensitive customer information and maintain the integrity of their systems.
An EIU survey of IT executives in the banking sector reveals that fraud detection is the top application of AI with almost 58% of banks using AI for this purpose. These systems can flag suspicious transactions and behaviors, such as unusual spending patterns or unauthorized access attempts, allowing banks to respond swiftly to potential fraud.
Credit scoring
Traditional credit scoring methods often rely on limited data and inflexible criteria like credit history, credit scores, and customer references, which can negatively influence credit decisions and exclude many potential borrowers. AI in banking technology can significantly improve credit scoring by including multiple data sources, such as social media activity, transaction history, and other non-traditional metrics.
AI models analyze these diverse customer data sets to evaluate creditworthiness more accurately, providing fairer and more inclusive lending opportunities. This approach helps banks make informed credit decisions at a fast pace and extend credit services to a broader population.
Risk management
Effective risk management is crucial for the stability and profitability of financial service providers. AI in banking can enhance risk management by predicting the potential impact of external risks such as currency fluctuations, political unrest, and natural disasters on the banking and financial institutions.
Generative AI advanced analytics and machine learning models can also forecast market trends, economic changes, and customer behaviors, enabling banks to make informed decisions.
AI-driven risk assessment tools evaluate the probability of loan defaults, market volatility, and other financial threats, allowing banks to accurately assess the risks and minimize losses.
Process automation
AI-driven process automation streamlines various banking operations, reducing manual effort and operational costs. Robotic Process Automation (RPA) empowered by AI can automate repetitive tasks such as data entry, transaction processing, and compliance checks. Moreover, it simultaneously learns from historical data and ongoing interactions to continuously improve the efficiency and accuracy of the bank's processes.
According to Autonomous, banks worldwide can reduce operational costs by 22% by 2030 with AI technologies.
By automating routine processes and repetitive tasks, banks can allocate resources to more strategic activities, allowing representatives to focus on building relationships with clients and improving overall productivity and customer service.
AML monitoring
Anti-Money Laundering (AML) compliance requires continuous monitoring and analysis of transactions to ensure that suspicious activities are promptly detected, preventing financial crimes.
AI in banking enhances AML monitoring by analyzing large volumes of transaction data and identifying suspicious patterns and relationships that may elude traditional rule-based systems. AI also reduces false positives, allowing organizations to focus on actual money laundering threats.
Regulatory compliance
The banking industry is one of the most highly regulated sectors in the world and is subject to strict regulatory requirements. Generative AI ensures regulatory compliance in financial services companies by automating data collection, analysis, and reporting processes.
Natural Language Processing (NLP) and deep learning models help compliance professionals interpret regulatory texts, improve their decision-making process, and ensure that the bank's practices align with current regulations.
AI-powered compliance management tools monitor transactions and identify non-compliance risks, reducing the burden on compliance teams and minimizing the risk of regulatory penalties.
Customer insights and segmentation
Generative AI enables banks to gain deeper insights into customer behavior and preferences through advanced data analysis. By analyzing transactional data, social media activity, and past customer interactions, generative AI models can segment customers based on their needs, preferences, and financial behaviors.
This segmentation allows banks to tailor their products, services, and marketing strategies to different customer groups, enhancing customer satisfaction and loyalty. Personalized recommendations and targeted offers driven by AI can significantly improve the customer experience and increase loyalty, ultimately boosting revenue for banks.
Find out more about the impact of AI on customer service in our article - AI in customer service.
Investment management
AI can assist in portfolio management, trading, and advisory services. AI algorithms analyze market sentiment, financial news, and historical trends to identify investment opportunities and risks. AI-powered tools provide personalized investment advice and generate risk profiles to help clients choose the most adequate investment products.
These AI-driven tools enable more precise wealth management, asset allocation, risk assessment, and portfolio optimization, helping investors achieve their financial goals.
Investment managers can also use AI and automation to obtain a large amount of data needed for environmental, social, and governance (ESG) scoring. This helps evaluate companies' sustainability practices, social impact, and corporate governance, enabling more informed and responsible investment decisions.
Chatbots
AI chatbots and virtual assistants can handle a wide range of customer queries, from account balances and transaction history to loan applications and fraud alerts. Available 24/7, chatbots provide instant responses and personalized customer support, improving customer satisfaction and reducing the workload on human agents.
Generative AI models powered by Natural Language Processing (NLP) enable chatbots to understand and respond to customer inquiries in natural language, making interactions seamless and natural. These chatbots can handle a wide range of customer questions, provide accurate information quickly, and maintain a conversational tone that is more human-like.
An advanced AI chatbot or virtual assistant can also automatically route complex issues to human agents, ensuring a smooth and comprehensive customer support experience. By effectively managing routine inquiries and escalating more complicated cases, AI chatbots contribute to the efficiency and effectiveness of customer support teams.
Predictive analytics
Predictive analytics, driven by AI, helps banks anticipate future market trends and customer behaviors. By analyzing historical data and identifying patterns, AI models can forecast customer needs, market changes, and potential risks.
Predictive analytics enables banks to make proactive decisions, such as offering personalized products, adjusting marketing strategies, exploring new sales opportunities, providing cross-sell offers, and managing risks more effectively. For example, predictive models can identify clients who are more likely to churn, allowing banks to implement customer retention strategies and improve customer loyalty.
In conclusion, AI has a huge impact on how banks operate in today’s digital reality, from cybersecurity and credit scoring to process automation and investment management. By leveraging generative AI, financial institutions can stay competitive, meet regulatory requirements, and provide better services to their customers.
Risks of AI in Banking
While AI offers numerous benefits to the banking industry, it also introduces several risks and challenges that financial institutions must address.
These include:
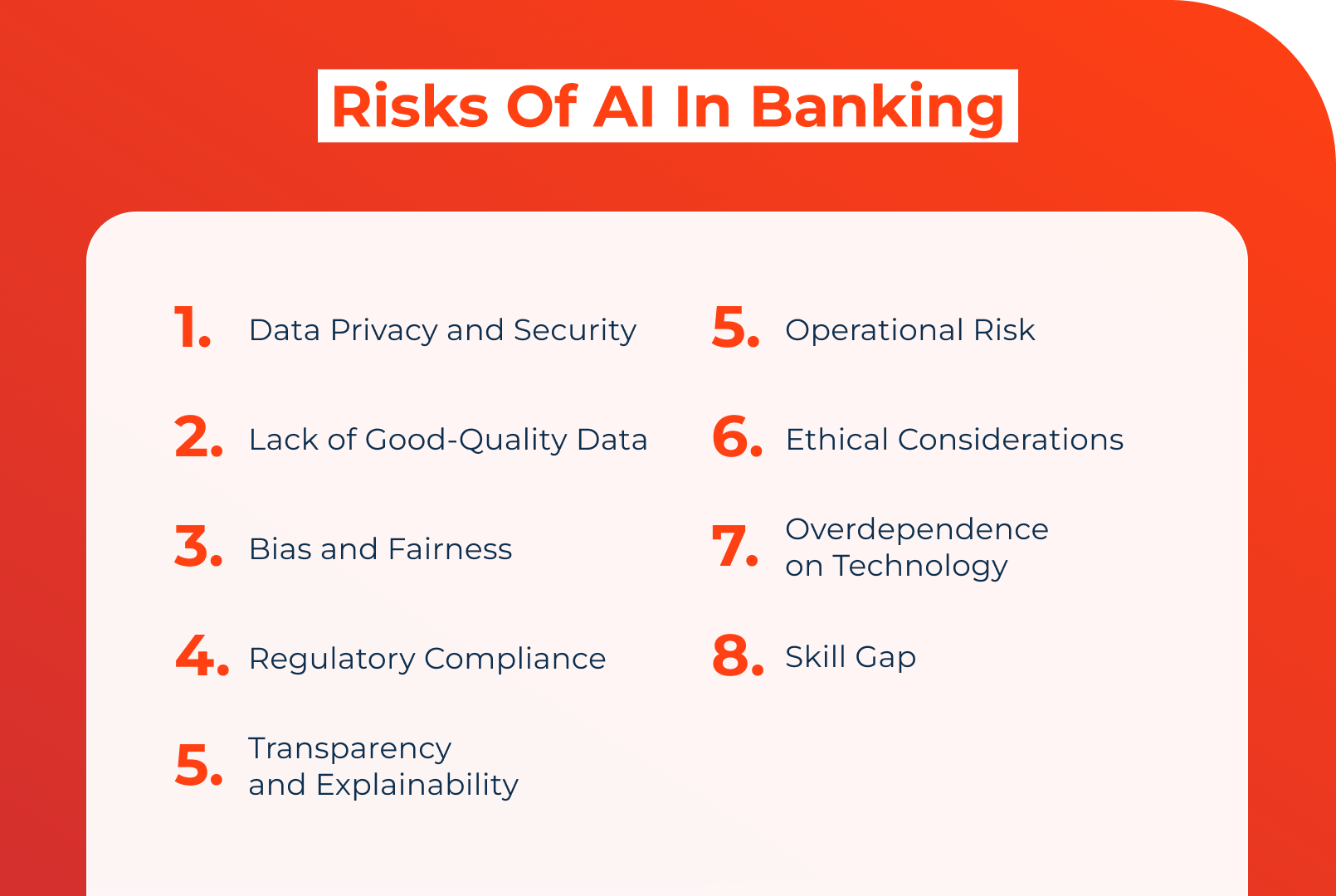
- Data privacy and security - AI handles vast amounts of sensitive information and ensuring the privacy and security of customer data is critical. Unauthorized access or data breaches can lead to significant financial losses and damage to the bank's reputation.
- Lack of good quality data - the effectiveness of AI systems heavily depends on the quality of the data they are trained on. Poor quality, incomplete, or inaccurate data can lead to unreliable AI outcomes, undermining the benefits and potentially causing harm.
- Bias and fairness - AI can unintentionally consolidate or even exacerbate existing biases in financial decision-making. If an AI model is trained on biased data, the decisions it makes can be unfair, leading to discrimination against certain groups of customers. This happened to Apple in 2019 when the AI-driven algorithm used by the company was accused of gender bias - allowing fewer credit lines to women than to men.
- Regulatory compliance - the dynamic nature of AI models can make it challenging to ensure compliance with evolving regulatory conditions. Financial institutions must ensure that their AI solutions adhere to all relevant laws and regulations, which can be complex and time-consuming.
- Transparency and explainability - many AI models, especially deep learning algorithms, operate as "black boxes," making it difficult to understand how they arrive at certain decisions. Lack of transparency and explainability can undermine trust and make it challenging to justify AI-driven decisions to regulators and customers.
- Operational risk - integrating AI into banking operations can introduce new operational risks, such as system failures or errors in AI models. These risks can disrupt banking services and lead to financial losses.
- Ethical considerations - the deployment of AI in banking raises various ethical issues, including concerns about surveillance, data use, and the potential for AI to make decisions that may not align with human values.
- Overdependence on technology - over-reliance on AI technology can make banks vulnerable to technology failures and cyber-attacks. Ensuring robust backup systems and disaster recovery plans is essential to mitigate this risk.
- Skill gap - the implementation of AI requires specialized skills that may not be readily available within the existing workforce. Banks need to invest in training and development to build the necessary expertise for managing AI technologies effectively.
Addressing these risks is crucial for banks to fully leverage the advantages of AI while safeguarding against potential downsides.
How to Implement AI in Banking
The adoption of generative AI in banking is growing rapidly, with over 85% of IT executives in banking having a clear strategy for adopting AI in the development of new products and services.
However, implementing AI in the banking sector requires a strategic and methodical approach to ensure successful digital transformation.
Below are key steps that banks should follow to implement AI effectively:
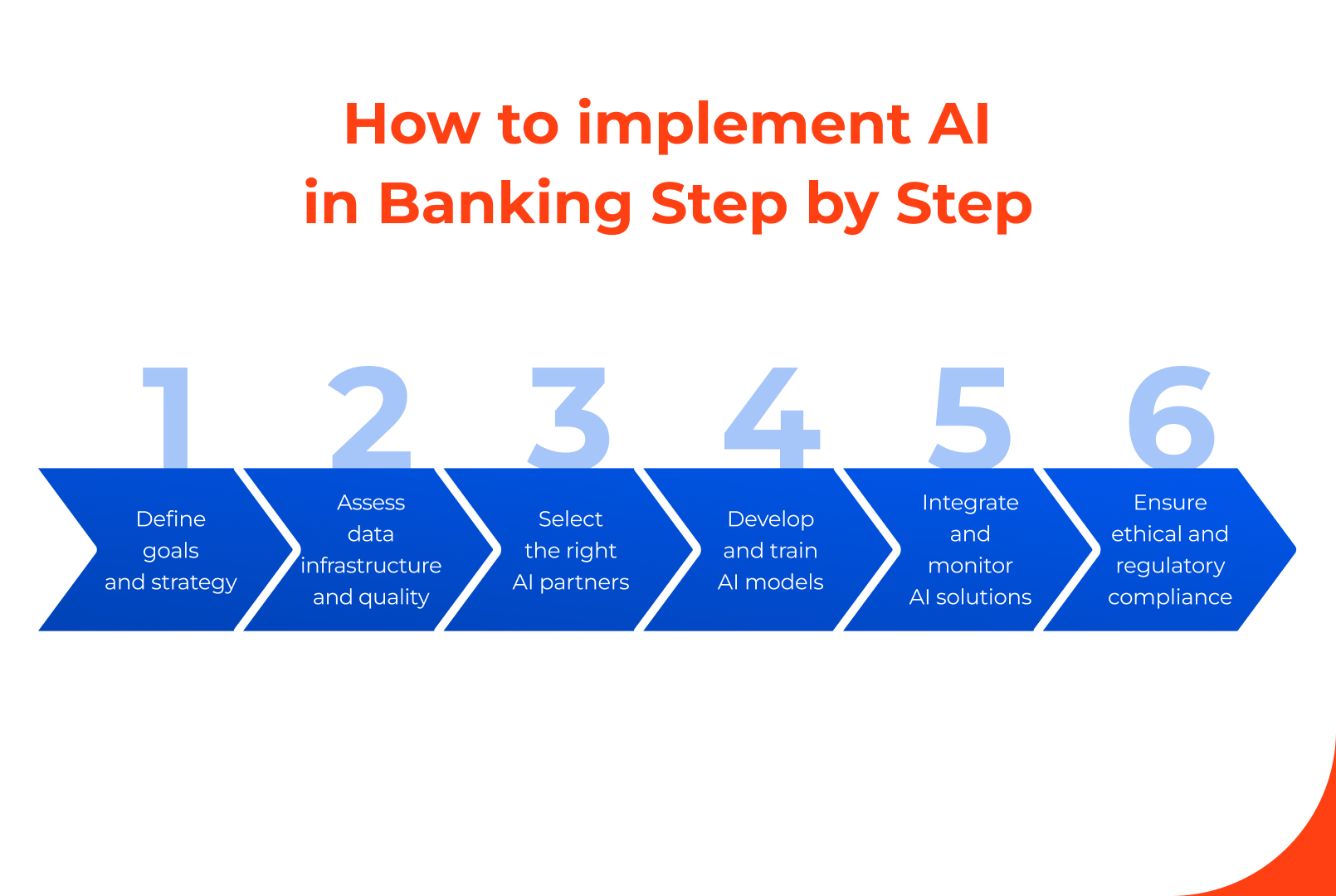
1. Define goals and strategy
The first step in implementing AI is clearly defining the goals and developing a comprehensive strategy. Banks should identify areas where AI can add value, such as enhancing customer service, improving risk management, or automating processes.
This involves:
- Setting clear goals - establishing what the bank aims to achieve with AI, such as reducing operational costs, improving customer satisfaction, enhancing fraud prevention, etc.
- Aligning with business strategy - ensuring that the AI initiatives align with the overall business strategy and goals of the bank.
- Stakeholder engagement - involving key stakeholders from different departments to gain insights and support for the AI implementation.
2. Assess data infrastructure and quality
AI relies heavily on high-quality data, so banks need to assess their existing infrastructure and address any gaps to ensure that the data used for AI is accurate, complete, and accessible.
This step includes:
- Data inventory - conducting a thorough inventory of available data sources, including customer data, transaction data, and external data.
- Data cleaning and integration - ensuring that the data is cleaned, standardized, and integrated into a central repository where it can be easily accessed and used by AI models.
- Data governance - establishing robust governance practices to ensure data privacy, security, and compliance with regulatory conditions.
3. Select the right AI partners
Choosing the appropriate AI partner is crucial for successful implementation. Banks should evaluate different AI tools and platforms to find those that best meet their needs.
This step involves:
- Vendor selection - partnering with reputable AI vendors that have expertise in the banking industry.
- Pilot programs - running pilot programs to test the selected AI technologies on a small scale before full-scale implementation.
4. Develop and train AI models
Once the right technologies and partners are in place, the next step is to develop and train AI models.
This involves:
- Model development - collaborating with data scientists and AI experts to develop models tailored to the bank’s specific needs.
- Training and validation - training the models using historical data and validating their performance to ensure accuracy and reliability.
- Improvements - continuously refining and improving the models based on feedback and new data to enhance their performance over time.
5. Integrate and monitor AI solutions
After developing and training the gen AI models, banks need to integrate them into their existing systems and processes.
This step includes:
- System integration - ensuring seamless integration of AI solutions with existing IT infrastructure, core banking systems, and customer-facing applications.
- Employee training - it’s necessary to provide relevant training and support to facilitate smooth adoption by employees.
- Monitoring and evaluation - real-time monitoring of the performance of AI is crucial to ensure they are delivering the expected outcomes. This includes setting up dashboards and KPIs to track the AI performance.
6. Ensure ethical and regulatory compliance
Throughout the implementation process, banks must ensure that their AI solutions comply with ethical standards and regulations.
This involves:
- Ethical considerations - addressing ethical issues related to AI, such as bias, transparency, and accountability.
- Regulatory compliance - ensuring that AI applications comply with financial regulations, data protection laws, and industry standards.
- Audit and review - conducting regular audits and reviews to identify and address any compliance issues.
Implementing AI in banking is a complex process that requires careful planning, execution, and continuous improvement. By following these steps, banks can effectively harness the power of generative AI to enhance their operations, improve customer experience, and stay competitive in the rapidly evolving financial landscape.
Banking AI With Creatio
The future of banking is bright with the integration of advanced AI technologies, revolutionizing the financial industry. In the coming years, AI will become even more sophisticated, enabling banks and financial institutions to offer hyper-personalized services, automate complex processes, and make data-driven decisions with greater accuracy.
AI will drive innovation in areas such as predictive analytics, enhanced cybersecurity and fraud detection measures, and real-time regulatory compliance. At the forefront of this transformation is Creatio with its Creatio.ai, a powerful tool designed to help banks leverage AI effectively.
Creatio.ai enhances customer engagement and helps build long-lasting relationships with clients by making highly personalized value propositions based on predictive scoring, AI/ML-powered next-best-offer intelligence, and clients' history of previous interactions. It also offers advanced analytics for deeper insights into customer behavior and market trends, enabling banks to make data-driven decisions and tailor their services to meet customer needs more effectively.
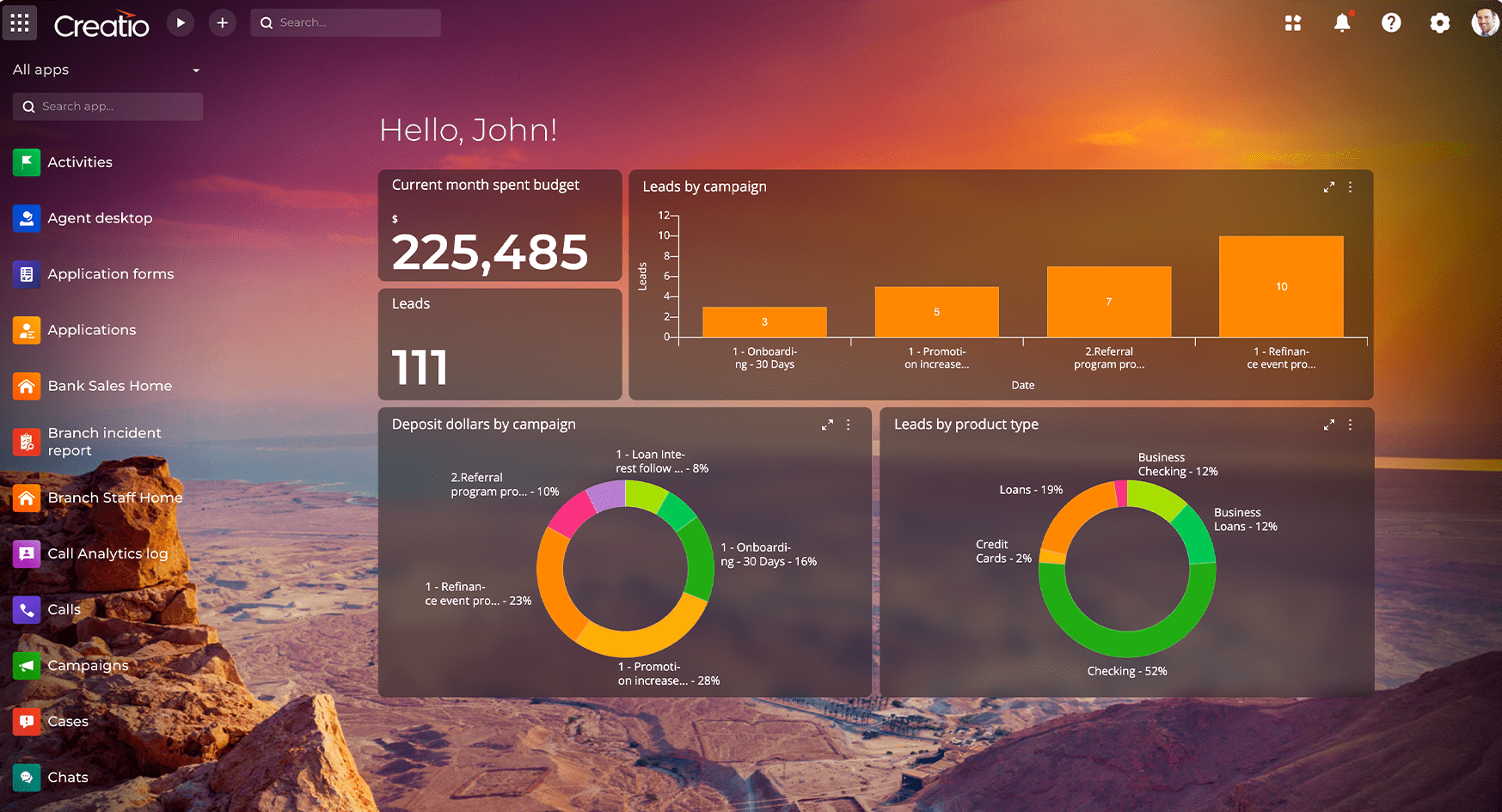
Creatio.ai automates routine tasks like loan application review/approval, customer identity verification, and account opening to improve productivity and reduce operational costs. It also helps maximize the efficiency of contact center operations with chatbots, and digital customer consultations, ensuring customers have access to timely and high-quality support around the clock.
Additionally, Creatio's AI aids in risk management and compliance by detecting fraud, assessing credit risk, and ensuring regulatory adherence. As a part of Creatio’s workflow automation and CRM platform, Creatio.ai enables banks to automate complex workflows and respond swiftly to market changes.
As AI continues to evolve, its impact on banking will become even more profound, with solutions like Creatio’s Copilot driving innovation, and enhancing digital transformation.
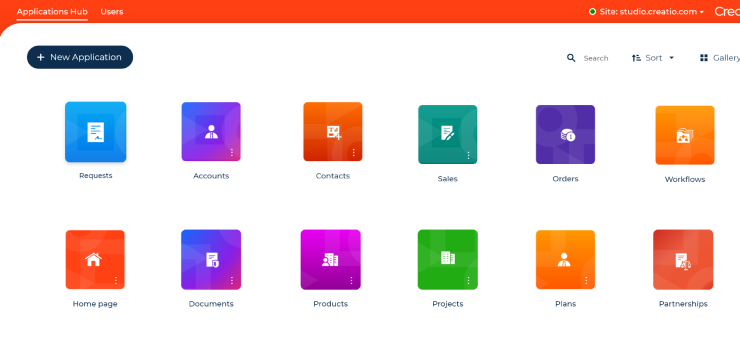