AI in Banking - How Artificial Intelligence Can Be Utilised in Banking Industry
Artificial Intelligence exerts a profound influence on the banking sector, contributing an estimated value of between 200 to 340 billion USD and boosting industry profits by as much as 15 percent, as reported by Statista. It serves as a catalyst for innovation, enhancing competitiveness, and enabling firms to secure a dominant position within the financial market.
According to The Economist, a remarkable 80% of senior banking executives are of the firm belief that the adoption of AI is pivotal to achieving success within the financial sector.
As AI technology continues its rapid evolution, its impact on banking operations becomes ever more pronounced, driving improvements in efficiency, security, and customer experience. This article delves into the multifaceted role of AI in banking, examining its diverse use cases, strategies for successful implementation, and the potential risks associated with its adoption.
What is AI in Banking?
Artificial Intelligence within the banking sector pertains to the deployment of advanced algorithms and machine learning methodologies to optimise internal operations and elevate customer-facing applications throughout the financial services industry. This cutting-edge technology aids in automating and refining processes encompassing customer service, fraud prevention, risk and investment management, as well as the provision of personalised financial advice.
Numeroues AI-driven tools are presently revolutionising the operational efficacy of the banking industry. AI-powered chatbots and virtual assistants are facilitating continuous, round-the-clock customer support, effortlessly managing routine inquiries and transactions. Concurrently, machine learning models analyse vast volumes of data to detect anomalies and prevent fraudulent activities. Moreover, AI-enhanced analytics deliver personalised financial insights and recommendations, significantly enhancing the customer experience.
According to Statista, the finance sector boasts one of the highest rates of Artificial Intelligence adoption across all industries. Through the judicious application of AI, banks are poised to augment productivity, reduce operational costs, offer more secure and refined services, and ultimately unlock a wealth of further benefits.
Artificial Intelligence Use Cases in Banking and Finance
The advent of AI holds tremendous promise for transforming the banking and finance sectors, offering an array of innovative solutions designed to enhance operational efficiency, bolster security, and elevate customer satisfaction.
Among the most prominent applications of AI within banking and finance are the following:
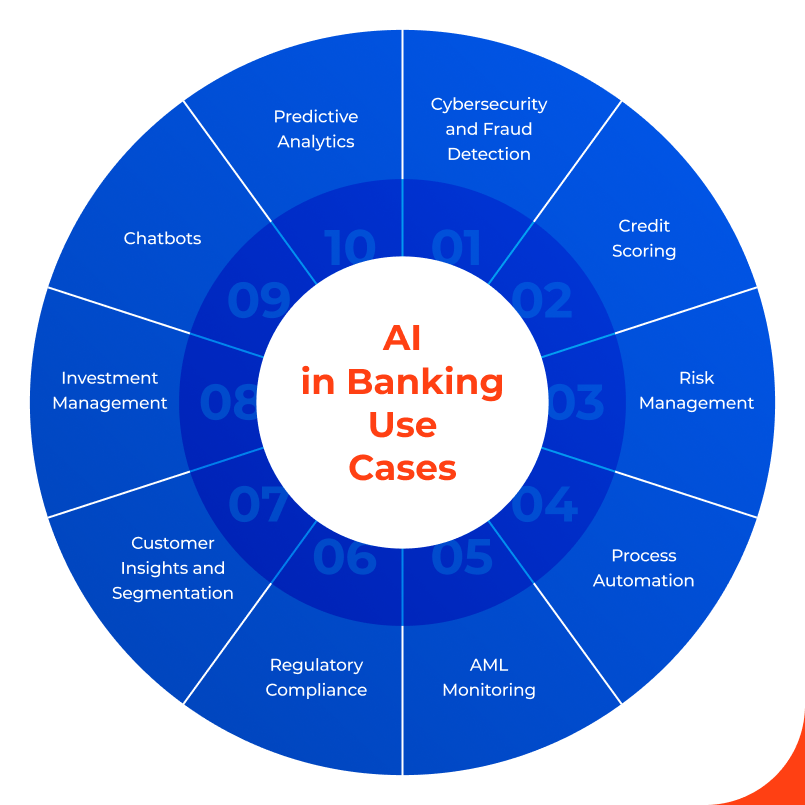
Cybersecurity and fraud detection
AI occupies a pivotal role in enhancing cybersecurity and advancing fraud detection mechanisms within the banking industry. AI-powered algorithms are adept at identifying anomalous patterns and irregularities in real time, facilitating the detection of potential threats with far greater speed and accuracy than traditional human methods.
These sophisticated systems process vast quantities of data to detect and neutralise threats such as malware and phishing attacks, constantly evolving in response to the ever-shifting landscape of cyber risks. By automating threat identification subsequent responses, AI assists financial institutions in safeguarding sensitive customer data and upholding the integrity of their digital infrastructures.
An EIU survey of IT executives within the banking sector reveals that fraud detection stands as the paramount application of AI, with nearly 58% of financial institutions using AI technologies for this very purpose. These intelligent systems are capable of flagging suspicious transactions and behaviors, such as anomalous spending patterns or attempts at unauthorised access, thereby enabling banks to take prompt and decisive action in mitigating potential fraud.
Credit scoring
Traditional credit scoring methodologies often hinge upon a narrow set of data and rigid criteria, such as credit history, numerical credit scores, and customer references. Such traditional approaches, while established, have the tendency to skew credit decisions unfavourably, excluding a considerable proportion of potential borrowers.
In contrast, the integration of AI within banking systems offers the potential to profoundly enhance credit scoring mechanisms by incorporating a far broader spectrum of data sources. These include, but are not limited to, social media activity, transaction history, and other unconventional metrics.
Through the application of AI-driven models, these diverse and multifaceted data sets are meticulously analysed, allowing for a far more accurate and comprehensive assessment of an individual’s creditworthiness. This, in turn, facilitates the provision of more equitable and inclusive lending opportunities. By embracing this innovative approachm banks are empowered to make well-informed, data-driven credit decisions with remarkable speed, thus expanding their reach and extending credit facilities to a much wider demographic.
Risk management
The imperative of effective risk management lies at the very core of ensuring both the stability and profitability of financial service providers. Within this context, the deployment of AI within the banking sector serves to augment risk management capabilities by forecasting the potential impact of external variables, such as currency fluctuations, political unrest, and natural disasters on the banking and financial institutions.
Advanced generative AI, coupled with sophisticated analytics and machine learning models, possesses the capability to anticipate market trends, economic shifts, and evolving customer behaviors, enabling banks to make informed decisions.
AI-driven risk assessment tools enable banks to delve deeply into the probabilities associated with loan defaults, market volatility, and other financial vulnerabilities. By evaluating these potential threats, banks can achieve a nuanced understanding of the risks at hand, enabling them to adopt pre-emptive measures to mitigate losses and ensure long-term resilience.
Process automation
AI-powered process automation serves as a transformative force within the banking sector, streamlining a plethora of operational activities while simultaneously reducing manual effort and associated operational expenditures. Robotic Process Automation (RPA), bolstered by AI, adeptly manages repetitive tasks, including data entry, transaction processing, and compliance verification. Furthermore, such systems possess the capacity to evolve continually by assimilating insights from historical data and ongoing interactions, thereby enhancing the precision and efficiency of banking processes over time.
According to a study conducted by Autonomous, the global banking industry stands poised to achieve a reduction in operational costs of up to 22% by the year 2030 through the adoption of AI technologies.
By delegating routine processes and monotonous tasks to AI-driven automation, financial institutions can reallocate valuable resources towards more strategic initiatives, enabling representatives to concentrate on fostering client relationships and augmenting overall productivity.
AML monitoring
Anti-Money Laundering (AML) compliance necessitates the perpetual scrutiny and evaluation of transactional activities to swiftly identify and mitigate any instances of suspicious behaviour, thereby safeguarding against financial crimes.
AI within the banking sector significantly augments AML monitoring by processing vast volumes of transactional data to uncover intricate patterns and anomalous relationships that might evade detection through conventional rule-based systems. Moreover, AI systems are adept at minimising false positive alerts, enabling financial institutions to allocate their focus more effectively on genuine threats of money laundering.
Regulatory compliance
Aso on of the world’s most regulated industries, the banking sector must adhere to a labyrinth of regulatory frameworks and statutory obligations. Generative AI facilitates the achievement of regulatory compliance by automating critical processes such as data collection, analysis, and reporting processes.
Natural Language Processing (NLP), coupled with deep learning algorithms, empower compliance professionals by providing nuanced interpretations of regulatory texts, thereby bolstering their decision-making processes and ensuring that institutional practices remain consistently aligned with evolving legal regulations.
AI-powered compliance management systems monitor transactional activity with unparalleled precision, proactively identifying potential risks of non-compliance. This not only alleviates the administrative burdens borne by compliance teams but also mitigates the prospect of incurring regulatory penalties, thereby reinforcing the institution’s commitment to operational integrity and adherence to legal standards.
Customer insights and segmentation
Generative AI empowers banking institutions to acquire sophisticated insights into customer behaviors and preferences by deploying advanced analytical methodologies across extensive datasets. By analysing transactional patterns, social media engagements, and historical customer interactions, generative AI models facilitate the nuanced segmentation of clientele according to their distinct need, preferences, and financial behaviors.
Such granular segmentation equips banks to devise bespoke products, services, and marketing strategies tailored to the unique customer groups, augmenting customer satisfaction and loyalty. Personalised recommendations and targeted offers, driven by AI-powered strategies, serve to elevate the overall customer experience and increase allegiance to the institution, thereby contributing to revenue augmentation.
Investment management
AI enhances the domain of investment management by facilitating advanced portfolio administration, trading strategies, and advisory services. AI algorithms analyse market sentiment, financial reportage, and historical trends to uncover lucrative investment opportunities, whilst simultaneously identifying potential risks.
AI-powered tools deliver bespoke investment advice tailored to individual client needs, generating detailed risk profiles to guide clients in selecting the most suitable financial instruments. Such AI-driven systems enable a higher degree of precision in wealth management, asset allocation, risk evaluation, and portfolio optimisation, thereby empowering investors to more effectively realise their financial ambitions.
Investment managers leverage AI technologies and automation processes to obtain a large amount of data requisite for Environmental, Social, and Governance (ESG) scoring. This capability allows for a thorough assessment of companies' sustainability initiatives, social impact, and corporate governance. Consequently, this data facilitates more informed responsible investment decision-making, aligning profitability with ethical imperatives.
Chatbots
AI-driven chatbots and virtual assistants are capable of managing an extensive array of customer queries, ranging from straightforward requests such as account balances and transaction histories to more intricate matters, including loan applications and fraud alerts. Available round the clock, these chatbots offer immediate, tailored responses, thereby enhancing customer satisfaction while alleviating the pressure placed upon human agents.
Generative AI models, powered by Natural Language Processing (NLP), enable these chatbots with the ability to comprehend and respond to customer inquiries in a manner that mirrors natural human language, thus fostering natural and intuitive interactions. Such systems are adept at addressing a broad spectrum of customer concerns, providing precise information with remarkable speed, all the while maintaining a conversational tone that approximates the human touch.
An advanced AI chatbot or virtual assistant possesses the capability to intelligently route more complex issues to human agents, ensuring that the overall customer support experience remains seamless and comprehensive. In this manner, by efficiently managing routine inquiries and escalating more complicated cases, AI chatbots significantly enhance the operational efficiency and effectiveness of customer support teams.
Predictive analytics
Predictive analytics, driven by AI, enables banks to anticipate forthcoming market trends and customer behaviors with precision. Through the analysis of historical data and the identification of recurring patterns, AI models are able to forecast shifts in consumer needs, market dynamics, and potential risks.
Predictive analytics enables banks to take proactive measures, such as offering personalised products, adjusting marketing strategies, exploring new sales opportunities, providing cross-sell offers, and managing risks more effectively. For instance, predictive models can identify clients who exhibit a heightened propensity to churn, thereby granting financial institutions the opportunity to implement targeted retention strategies, ultimately bolstering customer loyalty and satisfaction.
In conclusion, the influence of AI on the operational landscape of contemporary banking is profound, shaping everything from cybersecurity protocols and credit scoring methodologies to process automation and investment management strategies. By harnessing the power of generative AI, financial institutions are afforded a competitive edge, enabling them to navigate regulatory complexities while simultaneously delivering enhanced, more personalised services to clientele.
Risks Associated with the Implementations of AI in Banking
Whilst the integration of AI into the banking industry presents a plethora of transformative advantages, it simultaneously introduces a spectrum of risks and challenges that must be addressed by financial institutions to ensure responsible deployment.
Among the most pressing concerns are the following:
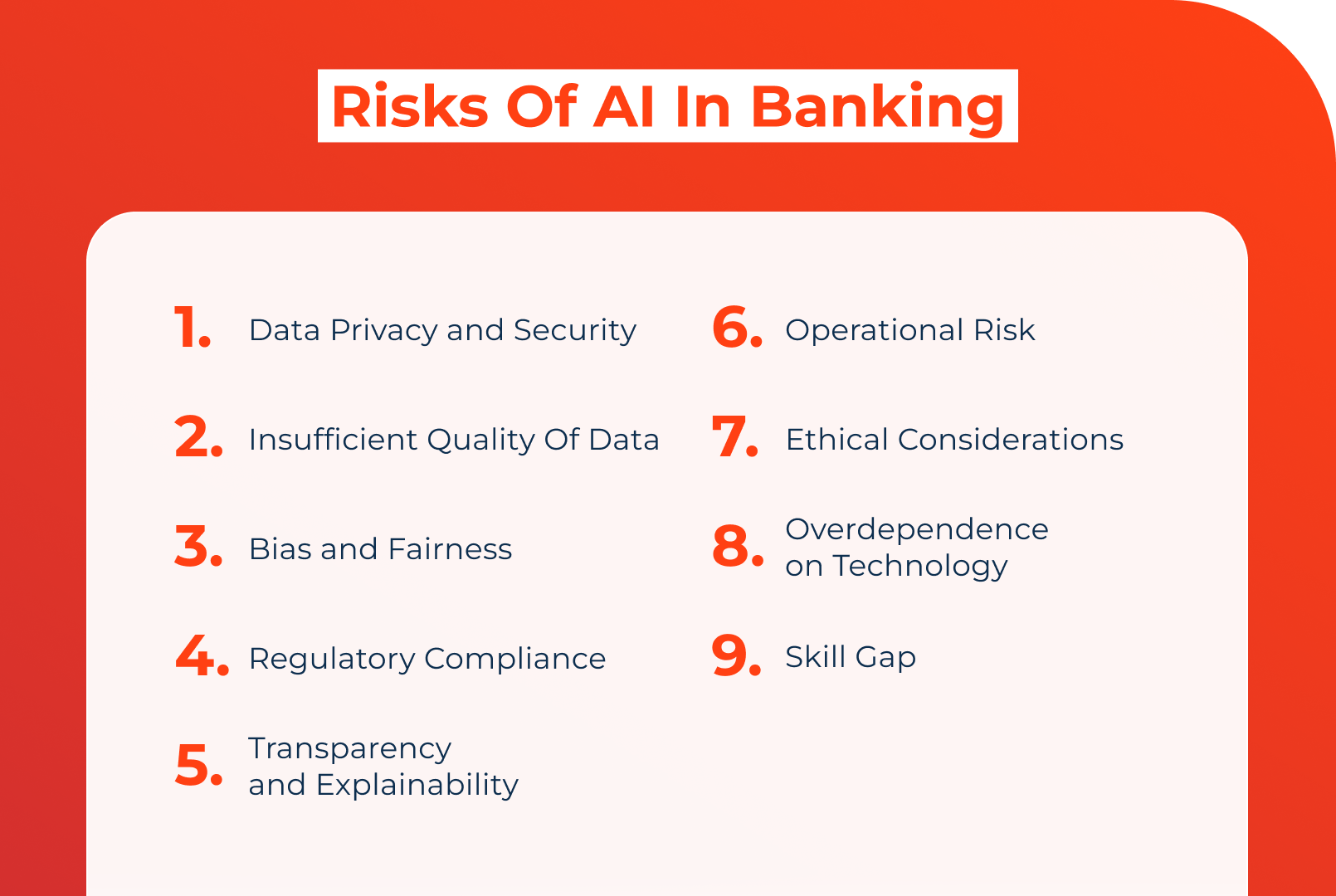
- Data privacy and security - AI systems necessitate the processing of vast quantities of sensitive and confidential customer information. Safeguarding the privacy and security of such data remains paramount, as any breach or unauthorised access could lead to significant financial losses, compromise customer trust and damage the bank's reputation.
- Insufficient quality of data - the effectiveness of AI-driven systems is intrinsically dependent upon the quality of the data they are trained on. Incomplete, inaccurate, or poor-quality data can lead to unreliable AI outcomes, undermining the benefits and potentially causing harm.
- Bias and fairness - the potential for AI to reinforce, or even amplify, existing biases in financial decision-making remains a critical concern. If an AI model is developed using biased data, the decisions it makes can be unfair, leading to discrimination against certain groups of customers. For instance, in 2019, Apple faced allegations of gender bias within its AI-driven credit assessment algorithm, which reportedly granted men more favourable credit limits than women. Such instances underscore the necessity of rigorous oversight and testing to ensure that AI operates equitably and inclusively.
- Regulatory compliance - the dynamic nature of AI models present a considerable challenge in ensuring adherence to evolving regulatory conditions. Financial institutions are tasked with the complex and often time-intensive responsibility of guaranteeing that their AI-driven solutions remain fully compliant with the plethora of applicable laws and regulations, which can be complex and time-consuming.
- Transparency and explainability - many advanced AI models, particularly those relying on deep learning algorithms, is their inherent opacity – often referred to as operation within a "black box". This lack of transparency renders it difficult, if not possible, for stakeholders to fully comprehend how specific decisions are made.
- Operational risks - incorporating AI into banking processes introduce operational risks, including the possibility of system failures or errors. Faulty AI models or inaccuracies in underlying data can lead to disruptions in services or incorrect decision-making. Such risks can result in financial losses and damage to the institution’s reputation.
- Ethical considerations - the implementation of AI within the banking sector gives rise to a plethora of ethical concerns, ranging from issues of surveillance and the responsibility of customer data to potential for AI to render decisions misaligned with societal values or human judgement.
- Overdependence on technology - an over-reliance on AI systems may render financial institutions vulnerable to technological malfunctions or cyber-attacks. Such dependencies highlight the necessity of establishing robust contingency measures, including comprehensive backup systems and disaster recovery strategies, to ensure resilience against unforeseen disruptions.
- Skill gap - the successful deployment of AI technologies require highly specialised skills that may not exist within the current workforce of many banking institutions. Addressing this skill gap demands significant investment in training programmes and professional development initiatives to cultivate the requisite knowledge and proficiency in AI management.
Addressing these risks is imperative for banks to harness the transformative potential of AI while ensuring the mitigation of any adverse consequences.
How to Implement AI in Banking
The adoption of generative AI within the banking sector is experiencing a market accelerations, as evidenced by the fact that over 85% of IT executives in the banking industry possessing a well-defined strategy for incorporating AI into the development of innovative products and services.
Nevertheless, the effective integration of AI technologies into banking sector operations necessitates a strategic and methodical approach, ensuring a seamless and impactful digital transformation that aligns with institutional objectives and customer expectations.
Outlined below are the fundamental steps that financial institutions ought to undertake in order to implement AI effectively:
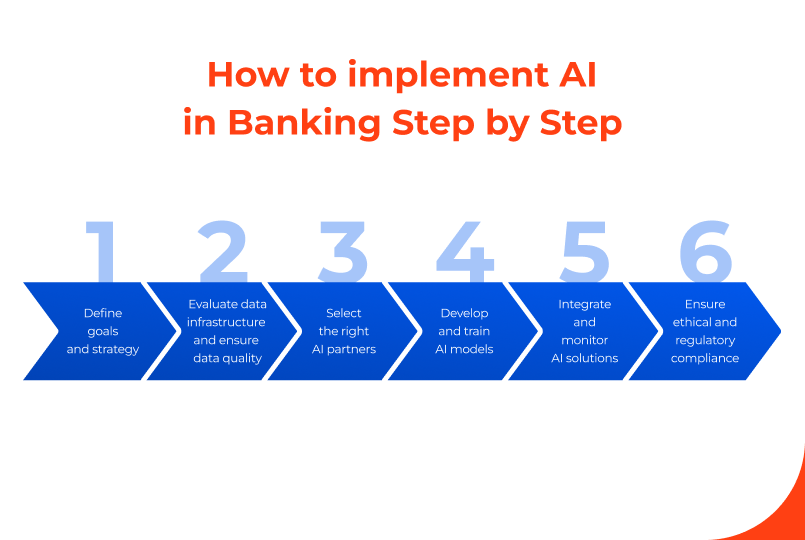
1. Define objectives and strategy
The initial steps in the integration of AI technologies necessitates the articulation of clearly defined objectives alongside the formulation of a detailed and cohesive strategy. Banks should pinpoint specific areas where AI can deliver value, such as elevating customer service standards, refining risk management processes, or streamlining operational workflows.
This involves:
- Establishing explicit objectives - banks must determine precise outcomes they seek to achieve through AI deployment, such as mitigating operational expenditures, improving customer satisfaction, or bolstering fraud detection.
- Ensuring strategic alignment - it is imperative that AI-driven initiatives are harmonised with the overall business strategy and goals of the bank.
- Stakeholder engagement - securing the involvement of key stakeholders across various departments is essential to gain insights, foster collaboration, and build support for the successful implementation of AI systems.
2. Evaluate data infrastructure and ensure data quality
Given that AI systems are fundamentally reliant on the availability and integrity of high-quality data, it is imperative for banking institutions to undertake a meticulous evaluation of their existing data infrastructure. Addressing deficiencies and ensuring that the data utilised for AI is both comprehensive and reliable forms the cornerstone of this process.
Key actions within this step include:
- Data inventory - undertaking a systematic review of all accessible data sources, encompassing customer profiles, transactional recordings, and external data.
- Data cleaning and integration - prioritising the standardisation, consolidation, and integration of disparate data into a centralised repository to facilitate seamless accessibility and optimal usability for AI-driven models.
- Data governance - implementing robust governance frameworks to uphold data privacy, fortify security, and ensure compliance with evolving regulatory requirements.
3. Select Appropriate AI partners
The selection of an optimal AI partner constitutes a pivotal element in ensuring the seamless and effective implementation of AI within banking operations. A thorough evaluation of available AI tools and platforms is necessary to identify those that align most closely with the institution’s bespoke requirements.
This process entails:
- Vendor selection - engaging with well-established AI vendors renowned for their expertise and demonstrable track record within the banking industry.
- Pilot programs - conducting pilot initiatives to trial the selected AI technologies on a manageable scale before full-scale implementation.
4. Develop and train AI models
With the appropriate technologies and collaborators secured, the subsequent step involves the development and refinement of AI models tailored to the bank’s operational needs.
Key activities in this phase involve:
- Model development - collaborating with data scientists and AI specialists to design and construct models tailored to the bank’s unique requirements.
- Training and validation - training the models using historical data and validating their performance to ensure accuracy and reliability.
- Improvements - establishing an iterative framework whereby models are continuously refined and optimised based on user feedback, evolving data inputs, and shifts in market conditions to ensure sustained performance enhancements over time.
5. Integrate and monitor AI solutions
Following the development and training of generative AI models, the subsequent task is their seamless integration into pre-existing systems and workflows.
This step includes:
- System integration - ensuring the harmonious embedding of AI solutions within legacy IT infrastructures, core banking systems, and customer-facing interfaces.
- Employee training - delivering tailored training initiatives to equip employees with the requisite skills for effective adoption and utilisation of AI tools.
- Monitoring and evaluation - establishing mechanisms for real-time monitoring, including dashboards and KPIs, to scrutinise AI performance and ensure alignment with anticipated outcomes.
6. Ensure ethical and regulatory compliance
The implementation of AI must be underpinned by adherence to rigorous ethical principles and regulatory frameworks to mitigate risks and maintain trust.
This necessitates:
- Ethical considerations - addressing ethical issues related to AI, such as bias, transparency, and accountability.
- Regulatory compliance - aligning AI applications with relevant financial regulations, data protection laws, and sectoral standards.
- Audit and review - conducting periodical audits and comprehensive reviews to identify and address any compliance issues.
Implementing AI within the banking sector constitutes a multifaceted endeavour necessitating meticulous planning, precise execution, and ongoing refinement. By adhering to these outlined steps, banks can effectively harness the transformative potential of generative AI, thereby optimising operational efficiencies, elevating the customer experience, and maintaining a competitive edge within the rapidly evolving financial landscape.
Banking AI With Creatio
The future of the banking industry is bright, underpinned by the integration of sophisticated AI technologies that are set to revolutionise financial services. In the years to come, AI will advance further, empowering banks to deliver hyper-personalised services, automate intricate processes, and execute data-driven decisions with greater accuracy.
AI will drive innovation across predictive analytics, fortify cybersecurity and fraud detection measures, and ensure real-time regulatory compliance. Leading this transformative wave is Creatio with its AI-powered Copilot – a robust solution designed to enable banks to harness AI effectively.
Creatio’s Copilot elevates customer engagement by fostering long-lasting relationships through highly personalised value propositions. These are driven by predictive scoring, AI/ML-powered next-best-offer intelligence, and an analysis of client’s previous interactions. It also offers sophisticated analytics for deeper insights into customer behavior and market trends, enabling banks to make data-driven decisions and tailor their services to meet customer needs more effectively.
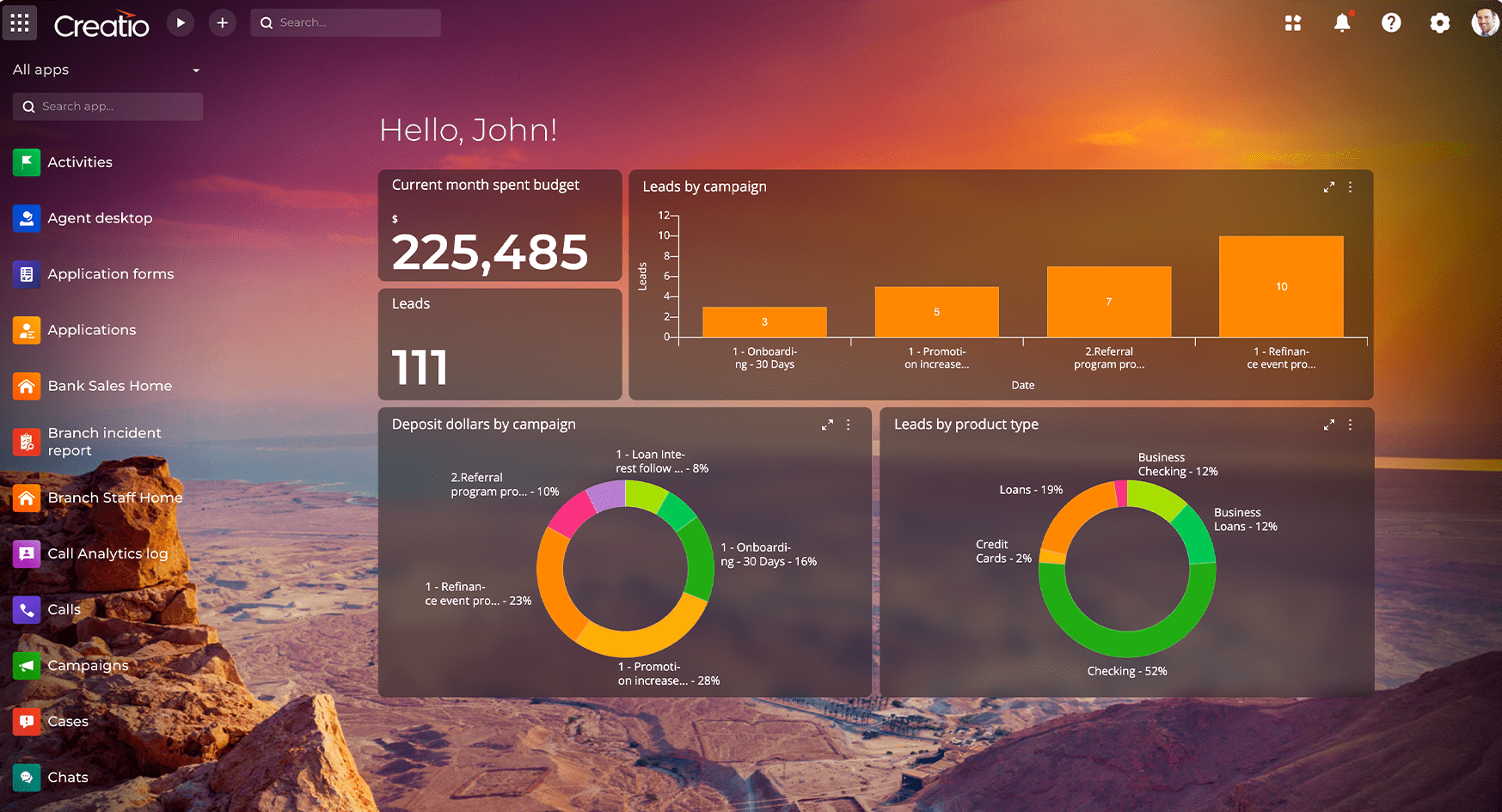
Copilot automates routine operations such as loan application review and approval, customer identity verification, and account opening, thereby enhancing productivity and reducing operational costs. It also optimises contact center efficiency through AI-powered chatbots and digital customer consultations, ensuring that clients receive timely, high-quality support around the clock.
Furthermore, Creatio's AI bolsters risk management and compliance by identifying fraud, evaluating credit risk, and ensuring adherence to regulatory standards. As an integral component of Creatio’s workflow automation and CRM platform, Copilot enables banks to automate complex workflows and respond swiftly to market changes.
As AI technology evolves, its impact on banking will become even more profound, with solutions such as Creatio’s Copilot driving innovation, and enhancing digital transformation.
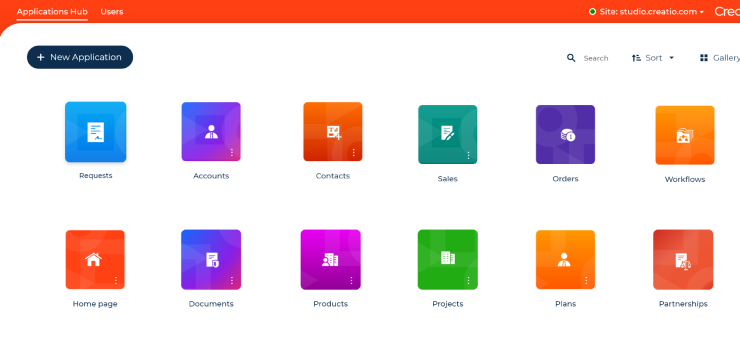