AI in Banking - How Artificial Intelligence Can Be Used in Banking Industry
Artificial Intelligence (AI) is reshaping the banking sector, delivering an estimated value of 317 AUD (~$200 USD) to 539 billion AUD (~$340 billion USD) while boosting industry profits by up to 15%, according to Statista. By driving innovations, enhancing competitiveness, and improving efficiency, AI enables financial institutions to maintain a leading edge in an increasingly competitive market.
The Economist reports that 80% of senior banking executives firmly believe AI is essential for success in the financial sector.
With its rapid evolution, AI continues to transform banking operations, delivering improvements in security, efficiency, and customer experience. This article explores the pivotal role of AI in banking, and the potential challenges businesses must navigate to make the most of its benefits.
What is AI in Banking?
AI in banking refers to the use of advanced algorithms and machine learning techniques to streamline internal operations and enhance customer-facing services across the financial sector. This innovative technology supports automation and improvement in areas such as customer service, fraud prevention, risk management, investment strategies, and personalised financial advice.
AI-driven tools are transforming how banks operate. AI-powered chatbots and virtual assistants provide 24/7 customer support, handling routine queries and transactions with ease. Machine learning models process large volumes of data to detect anomalies and prevent fraudulent activities. Additionally, AI-powered offer personalised financial insights and recommendations, delivering a more tailored and engaging customer experience.
Statista highlights that the finance sector is among the highest adopters of AI across industries. By leveraging AI, banks can boost productivity, cut costs, improve security, deliver more refined services, and unlock numeros other benefits in an increasingly competitive marketplace.
Artificial Intelligence Use Cases in Banking and Finance
AI is reshaping the banking and finance sectors, delivering innovative solutions to enhance efficiency, bolster security, and improve customer satisfaction.
Below are some of the key applications of AI in the industry:
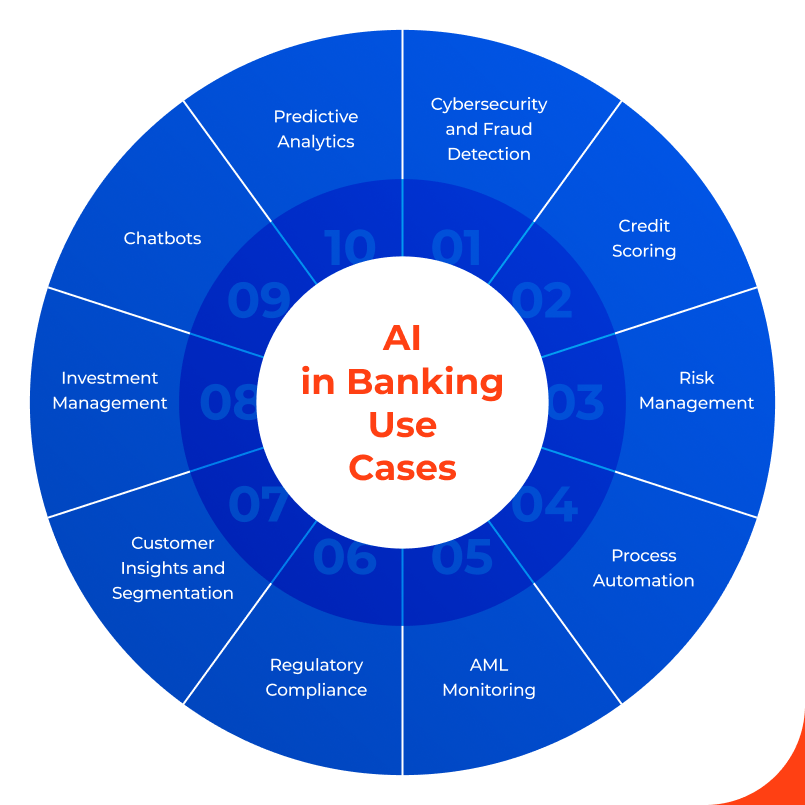
Cybersecurity and fraud detection
AI plays a crucial role in strengthening cybersecurity and advancing fraud detection in banking. AI-powered algorithms can detect unusual patterns and irregularities in real time, identifying potential threats with a speed and precision that far outpaces traditional methods.
These advanced systems process immense volumes of data to spot and neutralise threats like malware and phishing attacks. Constantly evolving alongside the shifting landscape of cyber risks, AI automates threat detection and response, helping financial institutions protect sensitive customer data and maintain the integrity of their digital systems.
A survey by the EIU found that fraud detection is the most significant application of AI in banking, with nearly 58% of financial institutions using AI for this purpose. Intelligent systems flag suspicious activities, such as unusual spending patterns or unauthorized access attempts, enabling banks to act swiftly to prevent potential fraud and safeguard customers’ assets.
Credit scoring
Traditional credit scoring methods rely on a limited range of data, such as credit history, numerical credit scores, and customer references. While these conventional systems are well-established, they often create barriers for many potential borrowers, excluding them from access to credit due to rigid criteria.
In contrast, AI in banking systems has the power to revolutionise credit scoring by tapping into a far broader array of data sources. These can include social media activity, transaction history, and other unconventional metrics, offering a deeper and more nuanced understanding of an individual’s financial behaviour.
AI-driven models analyse this rich, diverse data with precision, delivering a more accurate and inclusive assessment of creditworthiness. This enables banks to provide fairer lending opportunities and extend credit to a wider audience. By adopting AI-powered credit scoring, banks can make faster, data-driven decisions, expand their reach, and foster financial inclusion without compromising on risk management.
Risk management
Effective risk management is central to maintaining both the stability and profitability of financial service providers. In this space, AI is transforming the banking sector by enhancing risk management capabilities, helping institutions anticipate and prepare for external factors like currency fluctuations, political unrest, and natural disasters.
Generative AI, combined with advanced analytics and machine learning models, allows banks to predict market trends, economic changes, and shifting customer behaviors with greater accuracy. These insights empower financial institutions to make well-informed decisions that reduce exposure to potential risks.
AI-driven risk assessment tools dive deep into evaluating threats such as loan defaults, market volatility, and other financial vulnerabilities. By analysing these risks in detail, banks gain a clearer understanding of the challenges they face, enabling them to take proactive steps to minimise losses and build long-term resilience.
Process automation
AI-powered process automation is revolutionising the banking sector, simplifying countless operational tasks while cutting down on manual effort and operational costs. With the support of Robotic Process Automation (RPA) and AI, repetitive activities like data entry, transaction processing, and compliance checks are handled with ease. These systems continually improve by learning from historical data and ongoing interactions, boosting the accuracy and efficiency of banking operations over time.
Research from Autonomous highlights that the global banking industry could slash operational costs by as much as 22% by 2030 through the adoption of AI technologies.
By automating routine processes, financial institutions can free up resources to focus on strategic initiatives. This allows banking teams to dedicate more time to building stronger client relationships and driving overall productivity, ensuring long-term success in an increasingly competitive market.
AML monitoring
Anti-Money Laundering (AML) compliance requires constant oversight of transactional activities to swiftly detect and address suspicious behaviour, protecting against financial crimes.
AI significantly enhances AML efforts by analysing massive amounts of transactional data to uncover hidden patterns and unusual relationships that traditional rule-based systems might miss. Additionally, AI minimises false positive alerts, allowing financial institutions to focus their resources on genuine risks of money laundering.
Regulatory compliance
As one of the world’s most regulated industries, banking must navigate a complex web of regulatory requirements. Generative AI simplifie compliance by automating key processes like data collection, analysis, and reporting.
With the help of Natural Language Processing (NLP) and deep learning algorithms, compliance teams gain precise insights from regulatory texts, improving decision-making processes and ensuring adherence to evolving laws.
AI-powered compliance tools also monitor transactions with exceptional accuracy, flagging risks of non-compliance early. This reduces administrative strain, minimises the risk of regulatory penalties, and reinforces the bank’s dedication to operating with integrity and meeting legal obligations.
Customer insights and segmentation
Generative AI provides banks with a powerful tool for understanding customer behaviours and preferences by leveraging advanced analytics on large datasets. By examining transactional patterns, social media activity, and historical interactions, AI enables detailed segmentation of customers based on their specific need, preferences, and financial habits.
This detailed segmentation allows banks to design tailored products, services, and marketing strategies that resonate with different customer groups, boosting satisfaction and loyalty. Personalised recommendations and targeted offers powered by AI further enhance the customer experience, building stronger relationships and contributing to revenue growth.
Investment management
AI is revolutionising investment management by streamlining portfolio management, trading strategies, and advisory services. AI algorithms assess market sentiment, financial reports, and historical data to identify profitable investment opportunities, while also spotting potential risks.
AI-powered tools provide tailored investment advice, creating detailed risk profiles to help clients select the best financial options. These AI systems enhance wealth management, asset allocation, risk evaluation, and portfolio optimisation, empowering investors to better meet their financial goals.
Investment managers are also using AI to gather and analyse data for Environmental, Social, and Governance (ESG) scoring. This helps assess companies' sustainability, social impact, and governance practices, enabling more informed and responsible investment decisions that balance profitability with ethical considerations.
Chatbots
AI-driven chatbots and virtual assistants are becoming essential tools in managing a wide range of customer queries. From simple requests like checking account balances to more complex issues such as loan applications or fraud alerts, these systems offer 24/7 support with quick, personalised responses. This not only boosts customer satisfaction but also eases the load on human agents.
Powered by Natural Language Processing (NLP), generative AI models allow these chatbots to understand and respond in a way that feels natural and conversational, providing customers with accurate information quickly. These systems can handle a wide variety of issues, maintaining a friendly, human-like tone in the process.
Advanced AI chatbots or virtual assistants can also intelligently direct more complicated matters to human agents, ensuring the customer support experience remains smooth and efficient. By taking care of routine inquiries and passing on the more complex cases, AI chatbots greatly improve the effectiveness of customer support teams, helping businesses serve their customers better.
Predictive analytics
Ai-driven predictive analytics is transforming how banks forecast market trends and understand customer behavior. By analysing past data and recognising patterns, AI models can accurately predict shifts in consumer needs, market conditions, and potential risks.
With predictive analytics, banks can act proactively – offering personalised products, adjusting marketing strategies, exploring new sales opportunities, and managing risks more effectively. For example, predictive models can identify customers who are at risk of leaving giving banks the chance to implement targeted retention strategies and improve customer loyalty.
In conclusion, AI’s impact on modern banking is undeniable, influencing everything from cybersecurity and credit scoring to process automation and investment management. By adopting AI, financial institutions gain a competitive edge, allowing them to better navigate regulatory challenges while offering more personalised, efficient services to customers.
Risks of AI Implementation in Banking
While AI brings transformative benefits to the banking industry, it also introduces a range of risks and challenges that need to be carefully managed to ensure responsible use.
Here are some of the key concerns:
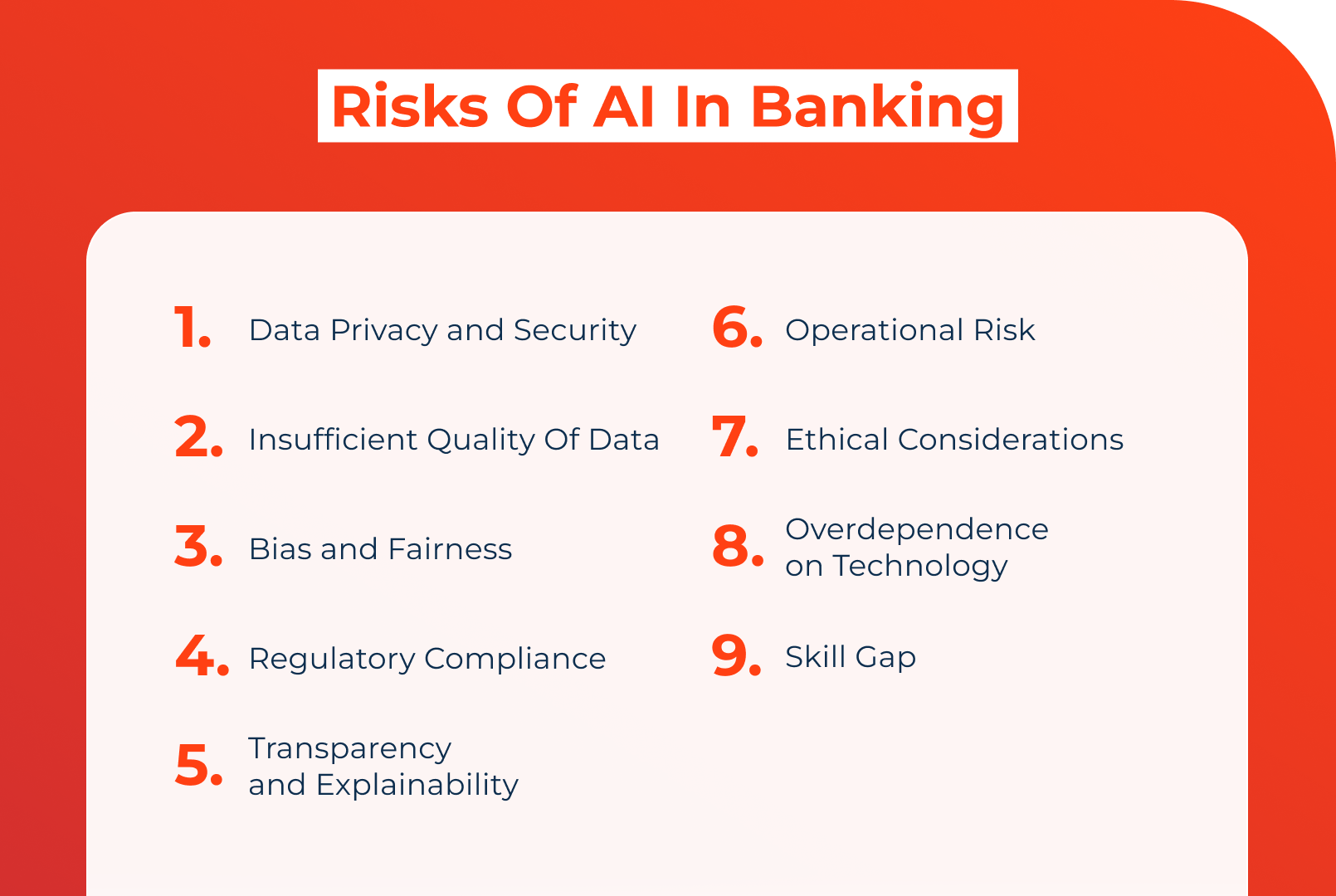
- Data privacy and security - AI systems rely on processing large amounts of sensitive customer data. Protecting the information is critical, as any breach or unauthorised access could result in significant financial losses, loss of customer trust, and reputational damage.
- Insufficient quality of data - AI effectiveness depends heavily on the quality of the data it’s trained on. If the data is incomplete, inaccurate, or of poor-quality, the AI’s outcomes may be unreliable, reducing its effectiveness and potentially causing harm.
- Bias and fairness - AI has the potential to reinforce or even amplify existing biases in decision-making. If an AI model is trained on biased data, its decisions can be unfair, discriminating against certain groups of customers. For example, in 2019, Apple faced allegations of gender bias in its AI-driven credit scoring, which reportedly resulted in men being offered higher credit limits than women. This highlights the need for strict oversight to ensure AI is used fairly and inclusively.
- Regulatory compliance - the constantly evolving nature of AI models makes it difficult for financial institutions to keep up with changing regulations. Ensuring that AI solutions comply with the complex and ever-changing legal landscape is a time-consuming and often complex task.
- Transparency and explainability - many advanced AI models, especially those using deep learning algorithms, operate in what’s known as a "black box". This means it can be hard, if not impossible, for stakeholders to understand exactly how certain decisions are being made. This lack of transparency raises concerns about accountability and trust.
- Operational risks - introducing AI into banking operations brings potential risks, such as system failures or errors. If AI models malfunction or are fed with inaccurate data, it can lead to disruptions in services or incorrect decisions, which can results in financial losses and damage to the institution’s reputation.
- Ethical considerations - AI’s role in the banking industry raises several concerns, including privacy issues, the handling of customer data, and the potential for AI to make decisions that may not align with societal values or human judgement.
- Overdependence on technology - relying too heavily on AI systems can make financial institutions vulnerable to technological failures or cyber-attacks. To safeguard against these risks, banks must implement strong contingency plans, including backup systems and disaster recovery strategies, to ensure resilience in the face of unforeseen disruptions.
- Skill gap - deploying AI effectively requires highly specialised skills, which many banks may not currently have in-house. To bridge this gap, financial institutions need to invest in training and professional development to build the necessary expertise in AI management.
By addressing these risks, banks can leverage AI’s transformative potential while ensuring they mitigate any negative impacts.
How to Implement AI in Banking
The use of generative AI in banking is gaining momentum, with over 85% of IT executives in the banking industry having a clear strategy for integrating AI into products and services. However, successful AI implementation requires a well-planned approach to ensure it aligns with business goals and enhances customer experiences.
Here are the key steps financial institutions should follow for effective AI integration:
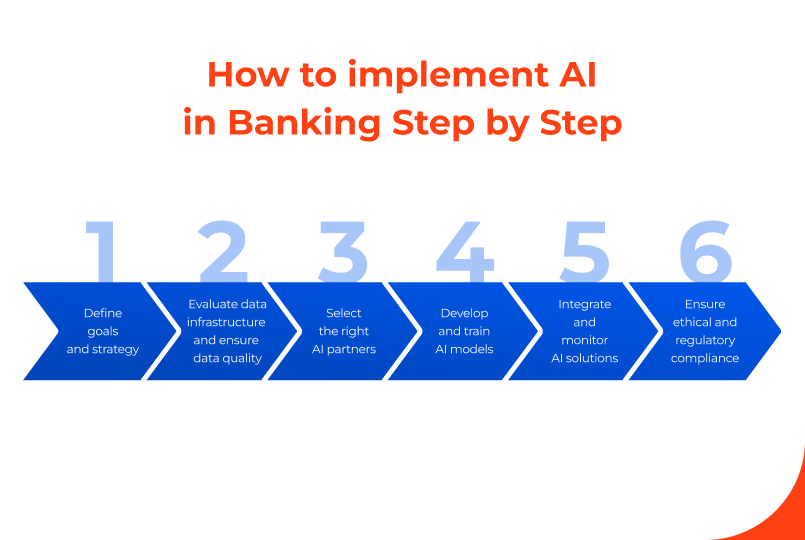
1. Define objectives and strategy
The first step in implementing AI is to clearly define your objectives and develop a strategic plan. Banks need to identify specific areas where AI can add value, whether it’s improving customer service, enhancing risk management, or optimising operations.
This involves:
- Setting clear goals - banks must establish what they hope to achieve with AI, such as reducing operational costs, increasing customer satisfaction, or improving fraud detection.
- Aligning with business strategy - it’s crucial that AI initiatives are in line with the bank’s overall business strategy and objectives to ensure a cohesive approach.
- Engaging stakeholders - involving key stakeholders across departments is vital to gather insights, encourage collaboration, and build support for the AI implementation process.
2. Evaluate data infrastructure and ensure data quality
Since AI systems rely heavily on accurate, high-quality data, it’s essential for banks to assess their current data infrastructure. Identifying gaps and ensuring that the data used for AI is both comprehensive and trustworthy is a key part of the process.
Key actions include:
- Data inventory - conduct a thorough review of all available data sources, including customer profiles, transactional records, and external data.
- Data cleaning and integration - focus on standardising, consolidating, and integrating data into a central system, making it easy to access and use for AI models.
- Data governance - implement strong data governance policies to ensure privacy, security, and compliance with changing regulations.
3. Select the Right AI partners
Choosing the right AI partner is key to a smooth and successful AI integration into banking operations. A careful assessment of available AI tools and platforms is necessary to ensure they meet the specific needs of the bank
This process involves:
- Vendor selection - partnering with reputable AI vendors known for their expertise and proven success in the banking industry.
- Pilot programs - running pilot projects to test the selected AI solutions on a smaller scale before rolling them out fully.
4. Develop and train AI models
Once the right technologies and partners are in place, the next step is to develop and fine-tune AI models that are customised to the bank’s specific needs.
Key activities during this phase involve:
- Model development - working closely with data scientists and AI experts to design models that meet the bank’s unique requirements.
- Training and validation - training the models with historical data and testing their performance to ensure they’re accurate and reliable.
- Improvements - setting up a system for ongoing model refinement, incorporating used feedback, new data, and market changes to ensure long-term effectiveness.
5. Integrate and monitor AI solutions
Once the AI models are developed and trained, the next step is to seamlessly integrate them into existing systems and processes.
This step includes:
- System integration - ensuring AI solutions work smoothly within existing IT infrastructures, core banking systems, and customer-facing interfaces.
- Employee training - offering tailored training programs to ensure staff have the skills needed to effectively use AI tools.
- Monitoring and evaluation - setting up real-time monitoring systems, like dashboards and KPIs, to track AI performance and ensure it’s meeting expected goals.
6. Ensure ethical and regulatory compliance
Implementing AI requires strict adherence to ethical standards and regulatory requirements to manage risks and maintain trust.
This involves:
- Ethical considerations - addressing ethical concerns around AI, like bias, transparency, and accountability.
- Regulatory compliance - ensuring AI solutions comply with relevant financial regulations, data protection laws, and industry standards.
- Audit and review - regular audits and reviews to spot and address any compliance issues.
Implementing AI in banking is a complex process that requires careful planning, precise execution, and continuous improvements. By following these key steps, banks can fully leverage the power of generative AI to boost operational efficiency, enhance customer experience, and stay competitive in the fast-evolving financial sector.
Banking AI With Creatio
The future of banking looks promising, driven by the integration of advanced AI technologies that are set to transform financial services. In the years ahead, AI will continue to evolve, allowing banks to deliver highly personalised services, automate complex processes, and make more accurate, data-driven decisions.
AI will drive innovation in areas like predictive analytics, enhance cybersecurity and fraud detection, and ensure real-time regulatory compliance. Leading the charge in this transformation is Creatio, with its AI-powered Copilot – a powerful solution designed to help banks make the most of AI.
Creatio’s Copilot boosts customer engagement by building long-lasting relationships through personalised offerings. It uses predictive scoring, AI/ML-powered next-best-offer intelligence, and an analysis of client’s previous interactions. Additionally, it provides advanced analytics for deeper insights into customer behavior and market trends, allowing banks to tailor their services more effectively.
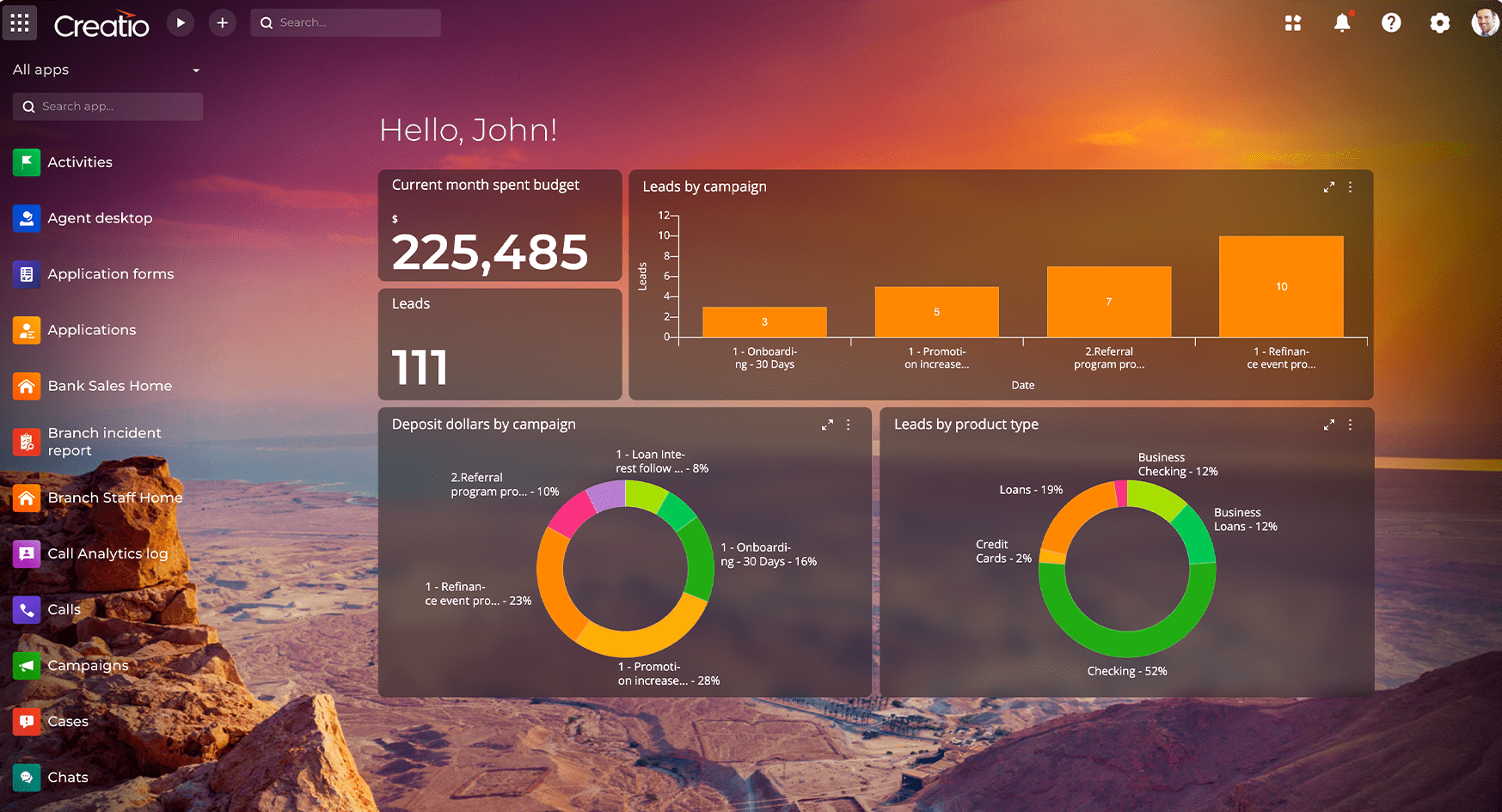
Copilot automates everyday tasks like loan application reviews, customer identity verification, and account opening, increasing productivity and reducing operational costs. It also enhances contact center performance with AI-powered chatbots and digital consultations, ensuring clients receive prompt, high-quality support at any time.
On top of that, Creatio's AI strengthens risk management and compliance by detecting fraud, assessing credit risk, and ensuring regulatory standards are met. As part of Creatio’s workflow automation and CRM platform, Copilot enables banks to streamline complex workflows and respond quickly to market changes.
As AI continues to evolve, its impact on banking will become even more profound, with solutions such as Creatio’s Copilot driving innovation, and enhancing digital transformation.
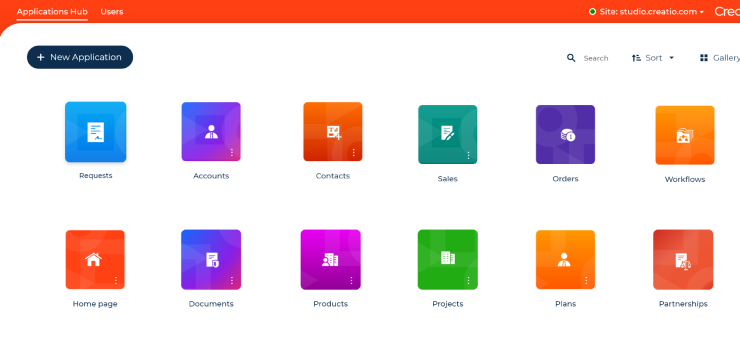